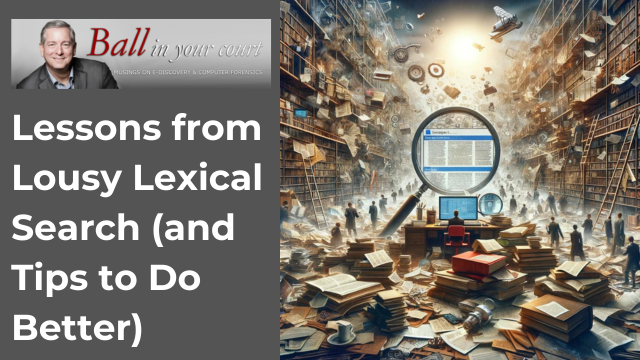
[EDRM Editor’s Note: The opinions and positions are those of Craig Ball. This article is republished with permission and was first published on February 26, 2024.]
Preparing a talk about keyword search, I set out to distill observations gleaned from a host of misbegotten keyword search efforts, many from the vantage point of the court’s neutral expert née Special Master assigned to clean up the mess. What emerged feels a bit…dark…and…uh…grouchy: like truths no one wants to hear about because then we might be compelled to change–when we all know how profitable it is to bicker about keywords in endless, costly rounds of meeting and conferring.
The problems I’m dredging up have endured for decades, and their solutions have been clear and accessible for just as long. So, why do we keep doing the same dumb things and expecting different outcomes?
In the 25+ years I’ve studied lexical search of ESI, I’ve learned that:
1. Lexical search is a crude tool that misses much more than it finds and leads to review of a huge volume of non-relevant information. That said, even crude tools work wonders in the hands of skilled craftspeople who chip away with care to produce masterpieces. The efficacy of lexical search increases markedly in the hands of adept practitioners who meticulously research, test and refine their search strategies.
2. Lawyers embrace lexical search despite knowing almost nothing about the limits and capabilities of search tools and without sufficient knowledge of the datasets and indices under scrutiny. Grossly overestimating their ability to compose effective search queries, lawyers routinely proffer untested keywords and Boolean constructs. Per Judge John Facciola a generation ago, lawyers think they’re experts in search “because they once used Google to find a Chinese restaurant in San Francisco that served dim sum and was open on Sundays.”
Without exception, every lexical search is informed and improved by the iterative testing of queries against a substantial dataset, even if that dataset is not the data under scrutiny. Iterative testing is invaluable when queries are run against representative samples of the target data. Every. Single. Time.
Craig D. Ball.
3. Without exception, every lexical search is informed and improved by the iterative testing of queries against a substantial dataset, even if that dataset is not the data under scrutiny. Iterative testing is invaluable when queries are run against representative samples of the target data. Every. Single. Time.
4. Hit counts alone are a poor measure of whether a lexical search is “good” or “bad.” A “good” query may simply be generating an outsize hit count when run against the wrong dataset in the wrong way (e.g., searching for a person’s name in their own email). Lawyers are too quick to exclude queries with high perceived hit counts before digging into the causes of poor precision.
5. A query’s success depends on how the dataset has been processed and indexed prior to search, challenging the assumption that search mechanisms just ‘work,’ as if by magic.
6. Lexical search is a sloppy proxy for language; and language is replete with subtlety, ambiguity, polysemy and error, all serving to frustrate lexical search. Effective lexical search adapts to accommodate subtlety, ambiguity, polysemy and error by, inter alia, incorporating synonyms, jargon and industry-specific language, common misspellings and alternate spellings (e.g., British vs. American spellings) and homophones, acronyms and initializations.
7. Lexical search’s utility lies equally in filtering out irrelevant data as it does in uncovering relevant information; so, it demands meticulous effort to mitigate the risk of overlooking pertinent documents.
Understanding some of these platitudes requires delving into the science of search and ESI processing. A useful resource might be my 2019 primer on Processing in E-Discovery; admittedly not an easy read for all, but a window into the ways that processing ESI impacts searchability.
Fifteen years ago, I published a short paper called “Surefire Steps to Splendid Search” and set out ten steps that I promised would produce more effective, efficient and defensible queries. Number 7 was:
“Test, Test, Test! The single most important step you can take to assess keywords is to test search terms against representative data from the universe of machines and data under scrutiny. No matter how well you think you know the data or have refined your searches, testing will open your eyes to the unforeseen and likely save a lot of wasted time and money.”
In the fullness of time, those ten steps ring as true today as when George Bush was in the White House. Then, as now, the greatest improvements in lexical search can be achieved with modest tweaks in methodology. A stitch in time saves nine.
Another golden oldie is my 2012 collection of ten brief essays called “Shorties on Search.”
But, as much as I think those older missives hold up, and despite the likelihood that natural language prompts will soon displace old-school search queries, here’s a fresh recasting of my tips for better lexical search:
Review any pre-processing steps applied, like normalization of case and diacriticals or use of stop words in creating the searchable indices.
Craig D. Ball.
Essential Tips for Effective Lexical Search in Civil Discovery
Pre-Search Preparation:
- Understand the Dataset
- Identify data sources and types, then tailor the search to the data.
- Assess the volume and organization of the dataset. Can a search of fielded data facilitate improved precision?
- Review any pre-processing steps applied, like normalization of case and diacriticals or use of stop words in creating the searchable indices.
- Know Your Search Tools
- Familiarize yourself with the tool’s syntax and keyword search capabilities.
- Understand the tool’s limitations, especially with non-textual data and large documents.
- Consult with Subject Matter Experts (SMEs)
- Engage SMEs for insights on relevant terminology and concepts.
- Use SME knowledge to refine keyword selection and search strategies.
Search Term Selection and Refinement:
- Develop Comprehensive Keyword Lists
- Include synonyms, acronyms, initializations, variants, and industry-specific jargon.
- Consider linguistic and regional variations.
- Account for misspellings, alternate spellings and common transposition errors.
- Utilize Boolean Logic and Advanced Operators
- Apply Boolean operators and proximity searches effectively.
- Experiment with wildcards and stemming for broader term inclusion.
- Iteratively Test and Refine Search Queries
- Conduct sample searches to evaluate and refine search terms.
- Adjust queries based on testing outcomes and new information.
Execution and Review:
- Provide for Consistent Implementation Across Parties and Service Providers
- Use agreed-upon terms where possible. The most defensible search terms and methods are those the parties choose collaboratively.
- Ensure consistency in search term application across the datasets, over time and among multiple parties.
- Sample and Manually Review Results
- Randomly sample search results to assess precision and recall.
- Adjust search terms and strategies based on manual review findings.
- Negotiate Search Terms with Opposing Counsel
- Engage in discussions to agree on search terms and methodologies.
- Document agreements to preempt disputes over discovery completeness.
- Make abundantly clear whether a non-privileged document hit by a query must be produced or whether (as most producing parties assume) the items hit may nevertheless be withheld after a review for responsiveness.
Post-Search Analysis:
- Validate and Document the Search Process
- Maintain comprehensive documentation of search terms, queries, exception items and decisions. Never employ a set of queries to exclude items from discovery without the ability to document the queries and process employed.
- Ensure the search methodology is defensible and compliant with legal standards.
- Adapt and Evolve Search Strategies
- Remain flexible to adapt strategies as case evidence and requirements evolve.
- Leverage lessons from current searches to refine future discovery efforts.
- Ensure Ethical and Legal Compliance
- Adhere to privacy, privilege, and ethical standards throughout the discovery process.
- Review and apply discovery protocols and court orders accurately.