Prompt Engineered, Edited and Quality Controlled by Ralph Losey. This essay was co-written by a human and machine with over 80% of the text “generated” by ChatGPT.


Active machine learning is a type of Artificial Intelligence that is used by attorneys to train a computer to find and classify documents relevant to a particular legal issue or lawsuit. The attorney provides the computer with a set of documents that have been identified as targets, such as documents that are relevant or privileged, and the computer uses this information to learn how to identify similar documents on its own. The goal of active machine learning is to automate the process of document review and classification, making it faster and more efficient for attorneys to locate and analyze relevant documents. Ralph Losey has created a whole online course to explain how to do it.
The target documents that are provided by lawyers to the computer for training are typically those that are relevant to a particular legal issue or lawsuit, or that have some other legal classification, such as privilege. The computer uses this information to learn how to identify similar documents on its own, so that it can assist the attorney in locating and analyzing relevant documents more efficiently.
Continuous, Intelligently Spaced Machine Training
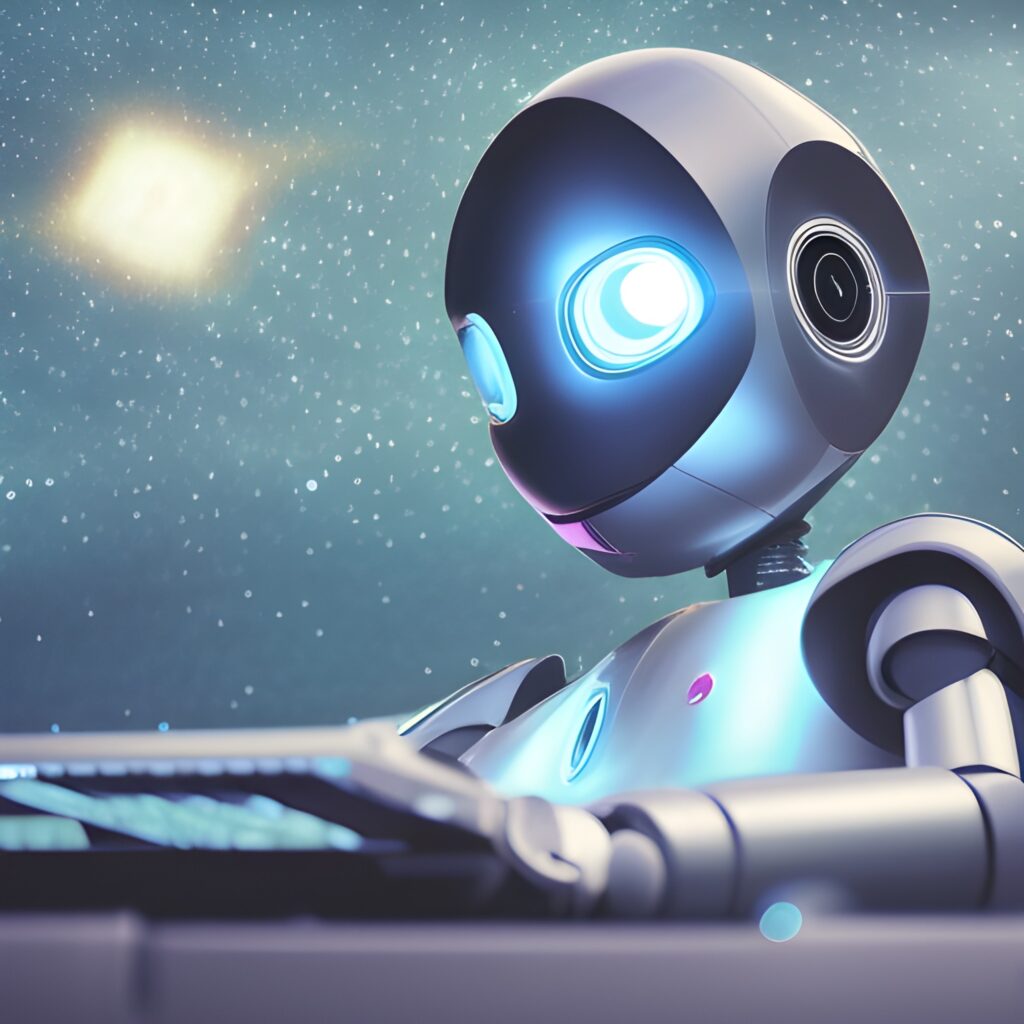
Continuous active learning is a machine learning approach in which the model is continuously updated with new data as it becomes available, rather than being trained on a fixed dataset and then deployed. This allows the model to continually improve its accuracy and performance over time. In the training system designed by Ralph Losey the AI Trainer spaces out the training sessions in time to fit the particular challenges of the project and data seen. Losey calls this Intelligently Spaced Training (IST). It is a training system for machine learning models that is designed to fit the circumstances of the case at hand. In this approach, the AI trainer spaces out the training sessions in time, rather than training the model continuously. This allows the trainer to selectively focus on particular aspects of the case and to test the machine’s effectiveness at different stages of the process.
In the context of legal document review, continuous active learning or Losey’s IST, are used to train a machine learning model to identify relevant documents. The attorney provides the model with a set of initial target documents, and then continuously update the model with new documents as they are discovered or become available. The model would use this new information to continually, or even better, intelligently, update and improve its ability to identify relevant documents.
The goal of IST Hybrid Multimodal Predictive Coding 4.0 is to optimize the learning process by allowing the machine to absorb and retain new information more effectively. This can be especially useful in the context of legal document review, where the volume of data can be large and the consequences of missing relevant documents can be significant. By allowing the machine to learn and test its effectiveness in a more structured and deliberate way, IST machine learning can help improve the accuracy and efficiency of the document review process.
One advantage of the ongoing training method is that it allows the model to adapt to changes in the data and remain up-to-date, which can be especially useful in a field like law where the relevant documents and legal issues may change over time, even during the document review project. You often start off with one definition of relevance and end up with another, more highly evolved understanding.
Hybrid Approach to Predictive Coding
There are several advantages to using a balanced hybrid approach that combines the efforts of both humans and computers in legal document review:

- Speed: Computers can process and analyze large amounts of data much faster than humans, so using a hybrid approach can help speed up the document review process.
- Accuracy: Humans are still generally better at tasks that require judgment, critical thinking, and understanding context, so a hybrid approach can help improve the accuracy of the document review process.
- Efficiency: By dividing the work between humans and computers, a hybrid approach can make the document review process more efficient. For example, humans could focus on the most important or complex documents, while the computer handles the more routine or straightforward ones.
- Cost-effectiveness: Using a hybrid approach can be more cost-effective than relying solely on human or computer review, as it allows the review process to be completed more quickly and with fewer errors.
- Improved collaboration: A hybrid approach can also facilitate better collaboration between humans and computers, as the two can work together to identify and analyze relevant documents more effectively.
Multimodal Approach to Predictive Coding
There are also advantages to a multimodal approach to predictive coding that combines AI search and all other types of search, including keywords and concept search. This is done in order to improve the accuracy and efficiency of the document review process. The basic kinds of search methods are shown in the pyramid. By using a combination of methods you can more effectively save on costs and implement a bottom line driven proportional strategy,

Some specific advantages of the multimodal approach include:
- Greater coverage: By using multiple search methods, a multi-modal approach can provide a more comprehensive search of the document set, increasing the chances of finding relevant documents.
- Improved accuracy: Different search methods can have different strengths and weaknesses, so using multiple search methods can help improve the overall accuracy of the search. This can be especially important in legal contexts, where the consequences of missing relevant documents can be significant.
- Reduced bias: Different search methods can also be subject to different types of bias. Using a multi-modal approach can help reduce the impact of these biases, as the different search methods may produce different results.
- Enhanced efficiency: By using multiple search methods, a multi-modal approach can help make the document review process more efficient. For example, humans could focus on reviewing the most relevant documents identified by the search, while the computer handles the less relevant ones.
- Improved collaboration: A multi-modal approach can also facilitate better collaboration between humans and computers, as the two can work together to identify and analyze relevant documents more effectively.
Advantages of a Hybrid Multimodal Approach to Predictive Coding
Some specific advantages of Ralph Losey’s methods and team approach to predictive coding include:

- Improved accuracy: By allowing the machine to learn and test its effectiveness in a more structured and deliberate way, Losey’s methods can help improve the accuracy of the document review process.
- Enhanced efficiency: The approach also helps make the document review process more efficient by allowing the machine to focus on the most important or complex documents, while humans handle the more routine or straightforward ones.
- Reduced bias: Losey’s multimodal methods can help reduce the impact of bias in the document review process by allowing the machine to learn from a more diverse and representative sample of documents.
- Improved collaboration: A hybrid, multi-modal, IST approach can also facilitate better collaboration between humans and computers, as the two can work together to identify and analyze relevant documents more effectively.
- Greater flexibility: Hybrid IST can also be more flexible than continuous active learning, as it allows the trainer to customize the learning process to fit the specific needs and circumstances of the case.
- Improved accuracy: By allowing the machine to learn and test its effectiveness in a more structured and deliberate way, IST can help improve the accuracy of the document review process.
- Enhanced adaptability: The IST approach to continuous active learning can also help the machine adapt to changes in the data or the legal landscape, as the trainer can update the training sessions as needed. This can help ensure that the machine remains up-to-date and relevant.
- Enhanced collaboration: The hybrid multi-modal IST approach also allows for better collaboration between humans and computers, as the trainer can work with the machine to identify and analyze relevant documents more effectively.
- Cost-effectiveness: Overall, the hybrid multi-modal IST approach can be more cost-effective than other methods, as it allows the review process to be completed more quickly and with fewer errors.
Chat-GPT’s “Easy Sales-type Language” Conclusion

The hybrid, multimodal, continuous active learning method is a dream team approach. It combines the speed and efficiency of computers with the judgment and critical thinking of humans, and it’s always learning and improving. This means that you get the best of both worlds: a super-accurate and up-to-date search that can find every last relevant document of importance, without missing a beat. Plus, because it’s continuously learning, it can adapt to changes in your case or the legal landscape, ensuring that you always have the most current and accurate information at your fingertips.
Using hybrid multimodal predictive coding search is like having a diverse team of genius attorneys, techs and paralegals working on your case. This super team can scour every nook and cranny of your document set, using every trick in the book (and a few that haven’t been invented yet) to find that crucial piece of evidence you need to win your case. Plus, they’re completely unbiased and super efficient, so you can save time and money while getting the best possible result. Who wouldn’t want them on their side?
Ralph Losey Copyright 2023 – ALL RIGHTS RESERVED