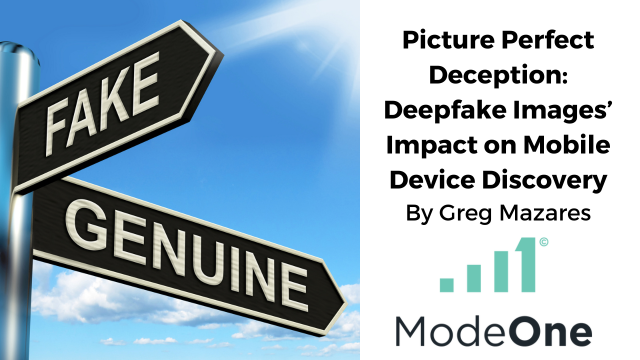
Deepfake images stored on cell phones can have significant implications for eDiscovery. Let’s examine some ways in which they may impact litigation, corporate compliance, and investigation activities and consider mitigation strategies.
Proving It’s Real
Deepfake technology can create incredibly realistic and convincing computer-generated images that are difficult to distinguish from genuine ones. This poses admissibility issues for parties involved in litigation when they rely on visual evidence to support their arguments. The authentication and verification of images become crucial concerns even as it becomes harder to determine whether an image has been manipulated or is genuine.
Making It Up
Inevitably, dishonest parties may try to use deepfake images to create false evidence to manipulate the legal system. If successful, these activities will impact the fairness and integrity of legal proceedings. Computer-generated or -altered images will also create more grounds for litigation – e.g., defamation and false claims suits against those who post them to social media sites or otherwise use them to harm others.
Outsmarting the AI
As deepfake technology advances, researchers and technologists are developing tools and techniques to detect and mitigate the spread of these misleading images. They can help identify signs of manipulation or provide statistical analysis to identify fake images. These tools range from standard digital forensics to AI-enabled image analysis software that analyze noise patterns, reverse engineering algorithms, or use statistical analysis to determine if an image is likely genuine or manipulated.
Here are a few of the most deployed image authentication techniques and technologies:
Digital Forensics
Digital forensics techniques can be used to analyze the authenticity and integrity of digital media, including images. Forensic experts examine the metadata, file structures, and other digital traces within an image to determine if it has been manipulated.
Facial and Image Analysis
Facial recognition and image analysis technologies identify anomalies in facial features or detect inconsistencies in lighting, shadows, or reflections. These techniques can help identify unnatural movements, glitches, or other telltale signs of a deepfake.
Machine Learning Algorithms
Machine learning algorithms are often employed to detect deepfake images. These algorithms analyze patterns, inconsistencies, and artifacts indicative of image manipulation. They can be trained on large datasets of real and false images to learn their characteristics and the differences between them.
The Power of Expertise
These technologies alone won’t solve the expanding challenge created by the growing popularity of computer-generated or -enhanced images. While proven eDiscovery processes already require technical experts to handle electronically stored information (ESI), the complexity and sophistication of the technologies used to identify deepfakes necessitate even greater technical skills and experience. Digital forensics professionals must develop an expert-level understanding of deepfake technology and the techniques used to analyze and detect manipulated images, then make decisions about the validity of the technology’s determinations.
Jerry Bui, a forensics expert and Managing Director at FTI Consulting Technology, noted: “Judges demand that the authentication of evidence occurs early during pretrial; you will see forensic experts used more frequently in response to FRE 902(14) to certify a key piece of evidence as genuine when it is likely to be challenged as a deepfake.”
Judges demand that the authentication of evidence occurs early during pretrial; you will see forensic experts used more frequently in response to FRE 902(14) to certify a key piece of evidence as genuine when it is likely to be challenged as a deepfake.
Jerry Bui, FTI Consulting Technology
Looking Forward
In the future, it might become standard operating procedure to employ forensics experts using specialized software to verify and validate the integrity of all images in a discovery data set. Additionally, legal professionals and eDiscovery experts should stay informed about the latest developments in deepfake detection technologies to meet competency requirements. They should develop image authentication strategies, collaborate with forensics technicians, and consider incorporating advanced detection and validation tools into their eDiscovery workflows. Additionally, courts may need to adapt their rules and procedures to account for deepfake evidence and establish guidelines for admissibility.
It is important to note that deepfake technology is constantly evolving and becoming more advanced, and detection tools and techniques are in a continuous race to keep up. As deepfake methods become more sophisticated, the detection systems must also be upgraded. The development and improvement of deepfake detection technologies are central to the ongoing delivery of justice.