[EDRM Editor’s Note: The opinions and positions are those of John Tredennick.]
As law firms and legal departments race to leverage artificial intelligence for competitive advantage, many are contemplating the development of private Large Language Models (LLMs) trained on their work product. While the allure of a proprietary AI system is understandable, this approach may be both unnecessarily complex and cost-prohibitive. Retrieval-Augmented Generation (RAG) systems offer a more practical, efficient, and potentially more powerful solution for law firms, legal departments and other organizations seeking to harness their collective knowledge while maintaining security and control.
Your Own Private LLM: Understanding the Appeal
Interest in private LLMs stems from compelling strategic considerations. Training an AI model on decades of carefully crafted legal documents, innovative solutions, and hard-won insights presents an attractive vision of institutional knowledge preservation and leverage. This appeal is rooted in several key factors that resonate deeply with law firm leadership.
First, private LLMs promise unprecedented control over training data. Firms can theoretically curate their training sets to include only their highest-quality work product, ensuring the model reflects their best thinking and preferred approaches. This level of control extends to writing style, analytical frameworks, and firm-specific procedures that distinguish the firm’s work in the marketplace.
Second, the customization potential of private LLMs suggests the ability to embed firm-specific expertise and methodologies directly into the AI system. This could include specialized knowledge in niche practice areas, proprietary deal structures, or unique litigation strategies that represent significant intellectual capital.
Third, security considerations weigh heavily in favor of private LLMs. The ability to maintain complete control over sensitive client information and proprietary work product within the firm’s own infrastructure appears to offer the highest level of data protection and confidentiality compliance.
The Hidden Challenges of Private LLMs
With these three benefits in mind, the reality of implementing and maintaining private LLMs presents a far more complex picture. These challenges manifest in several critical dimensions that firms must carefully consider.
The financial burden of private LLM implementation starts with substantial initial investment and training costs. Recent industry analyses suggest that these costs can be substantial–easily in the six figures range–incorporating expenses for data preparation, model development, infrastructure setup, and essential staffing. This includes the necessary recruitment of AI data scientists and specialized technical personnel—roles that command premium compensation in today’s market.
Ongoing operational expenses present another significant hurdle. Running a private LLM requires substantial computing resources, effectively demanding the operation of a small supercomputer. Annual infrastructure and staffing costs typically run into the hundreds of thousands to millions of dollars, significantly exceeding the operational costs of traditional legal technology systems.
Perhaps most critically, private LLMs face inherent limitations in maintaining currency with rapidly evolving legal developments and firm work product. Unlike traditional document management systems, LLMs cannot be simply “updated” with new content. Instead, they require complete retraining—a process that essentially means starting from scratch, incurring significant costs and computational overhead each time.
The performance gap between private LLMs and leading commercial models presents another serious consideration. Most private LLMs built on open-source foundations achieve performance levels comparable to GPT-3.5, while commercial models like GPT-4o and Claude 3.5 continue to advance rapidly. This creates a perpetual technology gap that becomes increasingly difficult to bridge, potentially leaving firms with expensive yet outdated AI capabilities.
The RAG System Alternative
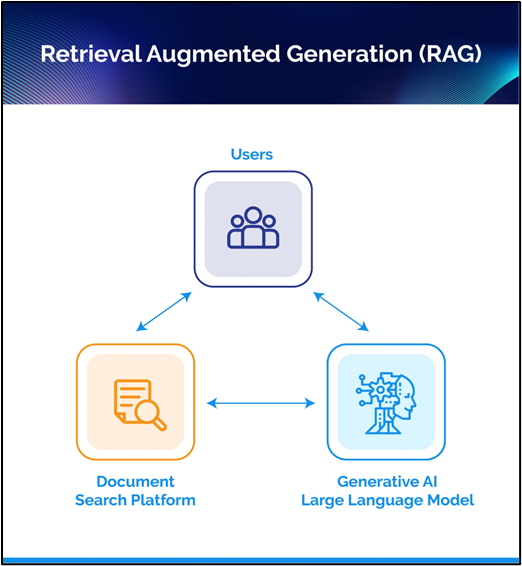
Retrieval-Augmented Generation (RAG) systems present a sophisticated, yet practical, alternative that addresses the core knowledge management needs of law firms and legal departments while avoiding the substantial drawbacks of private LLMs. By combining powerful commercial LLMs with intelligent document retrieval, RAG systems deliver immediate value while maintaining flexibility for future advancement.
At its core, a RAG system operates through a three-component architecture that elegantly solves the challenge of keeping AI responses current and accurate. The system begins with a sophisticated search platform that indexes and maintains the firm’s document repository. When a user poses a question, this platform identifies the most relevant documents from the firm’s collection, including the most recent work product. These documents are then presented to a state-of-the-art commercial LLM along with the user’s query, enabling the AI to generate responses grounded in the firm’s actual work product and expertise.
This architecture offers several distinct advantages over private LLMs. First, document currency is maintained through routine indexing rather than expensive model retraining. New work product becomes available for AI analysis as soon as it’s added to the system, typically through automated nightly updates. This ensures that responses always reflect the firm’s latest thinking and developments in the law.
Second, RAG systems leverage the most advanced commercial LLMs available, such as GPT-4o and Claude 3.5, providing significantly superior analytical capabilities compared to private models. As these commercial models continue to evolve and improve, RAG systems automatically benefit from these advancements without additional investment or technical overhead.
While private LLMs require months of development and training, RAG systems can be deployed in days or weeks, providing immediate value to the firm.
John Tredennick, CEO and Founder, Merlin Search Technologies.
Third, the implementation timeline and resource requirements for RAG systems are dramatically more favorable. While private LLMs require months of development and training, RAG systems can be deployed in days or weeks, providing immediate value to the firm. The technical expertise required for operation is also substantially lower, focusing on system configuration rather than complex model development and maintenance.
Security and Control in RAG Systems
Security concerns often drive firms toward private LLMs, but modern RAG systems offer equally robust—and in some cases superior—security controls while maintaining operational flexibility. These systems employ a multi-layered security approach that addresses the full spectrum of data protection requirements in legal practice.
The foundation of RAG system security lies in its single-tenant architecture. Each firm’s implementation exists in isolation, typically within a dedicated cloud environment that the firm can control. This approach provides complete data separation while maintaining the scalability and reliability benefits of cloud infrastructure.
Access control and encryption form the next critical security layer. Firms maintain ownership of their encryption keys and can implement granular access controls that reflect their organizational structure and client confidentiality requirements. Document access can be restricted by practice group, matter, or individual user, ensuring that sensitive information remains compartmentalized as needed.
Geographic deployment options address data sovereignty concerns, allowing firms to maintain compliance with regional privacy regulations. By selecting specific geographic regions for data storage and processing, firms can ensure alignment with client requirements and regulatory frameworks like GDPR or CCPA.
Modern RAG systems also provide comprehensive audit trails and security monitoring capabilities. Every interaction with the system can be logged and analyzed, providing transparency into system usage and helping firms maintain compliance with their ethical obligations regarding client data protection.
Implementation and Integration: A Strategic Approach
The implementation of any new technology in a law firm environment requires careful planning and execution. RAG systems offer distinct advantages in this regard, presenting a more streamlined path to operational value while minimizing disruption to existing workflows. Understanding the key elements of implementation helps firms make informed decisions and ensures successful adoption.
Deployment Timeline and Resource Allocation
The deployment timeline for a RAG system stands in stark contrast to private LLM implementation. While private LLMs typically require 6-12 months of development before producing any value, RAG systems can begin delivering results within days or weeks.
Technical Integration Considerations
RAG systems are designed to complement, rather than replace, existing legal technology infrastructure. Key integration points include:
- Document Management Systems: RAG platforms can interface with major legal DMS platforms, ensuring seamless access to current work product while maintaining existing security protocols and versioning systems.
- Knowledge Management Platforms: Rather than competing with existing knowledge management solutions, RAG systems enhance their value by providing an intelligent layer of access to stored information.
- Workflow Systems: Integration with practice management and workflow systems allows firms to embed AI-powered assistance at critical points in their legal processes, from research to document review and drafting.
Change Management and User Adoption
The success of any legal technology implementation depends heavily on user adoption. RAG systems present several advantages in this regard:
- Intuitive Interface: Users interact with the system through natural language queries, reducing the learning curve and encouraging adoption across different technological comfort levels.
- Immediate Value Demonstration: Unlike private LLMs, which require extensive training before showing results, RAG systems can demonstrate value from day one by providing accurate, context-aware responses to real-world queries.
- Graduated Implementation: Firms can begin with specific practice groups or use cases, allowing for controlled rollout and iterative improvement based on user feedback.
Resource Requirements
The resource requirements for RAG implementation are significantly more manageable than those for private LLMs:
Technical Expertise: While private LLMs demand specialized AI expertise, RAG systems primarily require standard legal technology skills for implementation and maintenance.
Training Resources: Training focuses on system utilization rather than model development, allowing firms to leverage existing legal technology training frameworks and personnel.
Infrastructure: Cloud-based deployment eliminates the need for extensive on-premises infrastructure, while utility-based pricing models allow firms to scale costs with actual usage.
Comparative Analysis: RAG Systems vs. Private LLMs
To facilitate informed decision-making, it’s essential to understand the key differences between RAG systems and private LLMs across critical operational dimensions. The following comparison chart illustrates these distinctions:
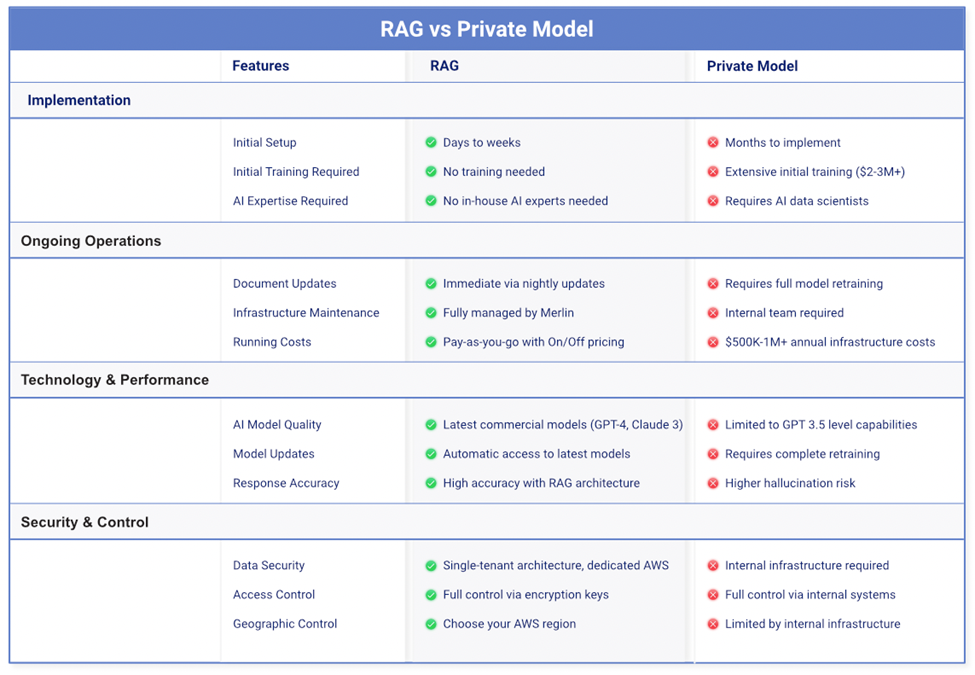
This comprehensive comparison reveals several crucial insights that law firms and legal departments should consider in their AI strategy:
Implementation and Expertise Requirements
The contrast in implementation approaches is striking. While RAG systems can be operational within days or weeks, private LLMs demand months of development and millions in initial investment. More significantly, the expertise requirements differ fundamentally. Private LLMs necessitate specialized AI data scientists—a scarce and expensive resource—while RAG systems can be managed with existing legal technology expertise supplemented by vendor support.
Operational Efficiency and Cost Structure
The operational models present perhaps the most significant divergence. RAG systems offer immediate access to new documents through automated updates, while private LLMs require complete retraining to incorporate new information—a process that is both costly and time-consuming. The pricing models also differ substantially: RAG systems typically offer utility-based pricing with the ability to control costs through usage management, while private LLMs require substantial fixed infrastructure investment regardless of usage levels.
Technology Currency and Performance
RAG systems maintain technological currency by leveraging the latest commercial LLMs, automatically benefiting from advances in AI capabilities without additional investment. In contrast, private LLMs typically operate at a GPT-3.5 level of capability, with improvements requiring substantial reinvestment in training and infrastructure. This technology gap becomes increasingly significant as commercial LLMs continue their rapid advancement.
RAG systems maintain technological currency by leveraging the latest commercial LLMs, automatically benefiting from advances in AI capabilities without additional investment.
John Tredennick, CEO and Founder, Merlin Search Technologies.
Security Architecture and Control
While both approaches offer security controls, they implement them differently. RAG systems provide security through single-tenant architecture and dedicated AWS environments, offering both isolation and flexibility. Private LLMs rely on internal infrastructure, which may appear more controlled but often lacks the sophisticated security features and regular updates available in enterprise cloud environments.
This comparative analysis underscores why many firms are concluding that RAG systems offer a more practical, cost-effective, and future-proof approach to leveraging AI in legal practice. The combination of lower implementation barriers, superior operational flexibility, and access to cutting-edge AI capabilities presents a compelling value proposition for firms seeking to enhance their knowledge management capabilities.
What about Enterprise LLM Security?
Many firms initially questioned the security of public LLMs like GPT and Claude, particularly after ChatGPT’s public beta reserved rights to use submitted data for training. However, enterprise LLM implementations now provide comprehensive security through contractual and architectural safeguards.
Major providers like Microsoft, OpenAI, and Anthropic offer enterprise agreements with specific legal data protections:
- All communications remain private and temporary
- No data retention or model training use
- Information exists only in the momentary context window
- Complete isolation from other users’ data
Perhaps the most important thing to understand is this: LLMs can’t remember or share the data you send to them, regardless of whether they are public or private. To the contrary, the data you send for analysis typically stays with the LLM for about the time it takes the LLM to respond. Further requests to the LLM must include the previous information in your conversation.
For comparison, consider the email and other files organizations regularly store with a cloud provider such as Microsoft or Google. That data, which includes client and organization confidential information, can reside on cloud servers for months or years. As a practical matter, the milliseconds of context window exposure with an LLM present significantly less risk than conventional document management systems.
Making the Strategic Choice: A Framework for Decision
The decision to implement any AI system requires careful consideration of multiple factors beyond pure technological capabilities. Law firms and legal departments must evaluate these solutions within the broader context of their strategic objectives, ethical obligations, and professional responsibilities.
Strategic Alignment
Begin by assessing how each approach aligns with your firm’s strategic goals:
Knowledge Management Objectives: Consider how effectively each solution supports the capture, preservation, and leverage of institutional knowledge. RAG systems excel at making current knowledge immediately accessible, while private LLMs may offer deeper but less current integration of historical information.
Client Service Impact: Evaluate how each solution enhances client service delivery. The ability to quickly access and apply firm expertise can significantly improve response times and service quality. RAG systems’ currency and accuracy advantages often translate directly to superior client outcomes.
Competitive Positioning: Consider how each approach positions your firm in an increasingly technology-driven legal market. While private LLMs might appear more prestigious, RAG systems’ superior capabilities and cost-effectiveness often provide greater competitive advantage.
Risk Assessment
Conduct a thorough risk assessment of each approach:
Technical Risk: Private LLMs carry significant technical risks related to development success, ongoing maintenance, and technological obsolescence. RAG systems minimize these risks through proven architecture and continuous updates to underlying commercial models.
Operational Risk: Consider the impact of system failures or performance issues. RAG systems’ modular architecture provides inherent redundancy and easier troubleshooting, while private LLMs present single points of failure that could impact firm operations.
Financial Risk: Evaluate the financial implications of each approach, including both initial investment and ongoing costs. RAG systems’ utility-based pricing models offer greater financial flexibility and risk mitigation compared to the substantial fixed costs of private LLMs.
Return on Investment
Calculate ROI across multiple dimensions:
Quantitative Metrics:
- Implementation costs and timelines
- Ongoing operational expenses
- Time savings in knowledge retrieval and application
- Reduction in duplicate work
- Improved matter staffing efficiency
Qualitative Benefits:
- Enhanced knowledge sharing across practice groups
- Improved junior attorney development
- More consistent work product
- Better preservation of institutional knowledge
- Increased client satisfaction
Conclusion: Charting the Path Forward
As law firms and legal departments navigate the integration of AI into their practice, the choice between private LLMs and RAG systems represents a critical strategic decision. The evidence strongly suggests that RAG systems offer a more practical, cost-effective, and capable solution for most firms. Their combination of immediate value delivery, superior technology currency, and manageable implementation requirements provides a compelling advantage over private LLM alternatives.
However, successful implementation requires more than just selecting the right technology. Consider these key success factors:
- Clear Strategic Vision: Develop a clear understanding of how AI will enhance your firm’s practice and competitive position.
- Thoughtful Implementation Planning: Create a detailed implementation plan that addresses technical, operational, and change management requirements.
- Stakeholder Engagement: Ensure early involvement of key stakeholders, from practice group leaders to IT professionals and knowledge management specialists.
- Measured Rollout Strategy: Begin with targeted implementations that can demonstrate clear value before expanding to broader applications.
- Continuous Evaluation and Adaptation: Maintain regular assessment of system performance and user adoption, adjusting approach based on feedback and emerging needs.
For firms ready to move forward with AI integration, RAG systems offer a pragmatic path to capturing the benefits of artificial intelligence while minimizing risk and maximizing return on investment. The technology has matured to a point where the question is no longer whether to implement AI, but how to implement it most effectively. RAG systems provide an answer that balances ambition with practicality, innovation with reliability, and technological sophistication with operational simplicity.
Assisted by GAI and LLM Technologies per EDRM GAI and LLM Policy.