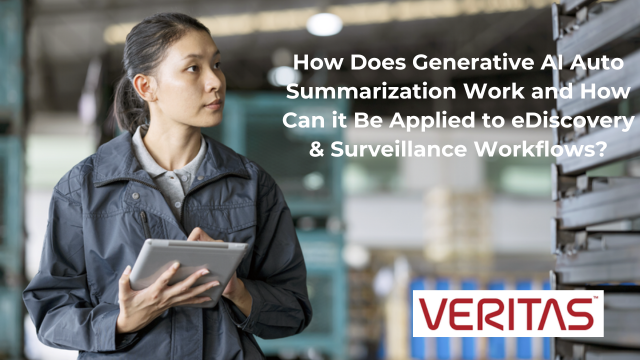
[EDRM Editor’s Note: EDRM is happy to amplify our Trusted Partners news and events. The opinions and positions are those of Veritas and Irfan Shuttari. This article was first published on May 21, 2024].
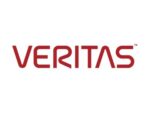
It seems everyone is talking about generative AI and its capabilities these days. Many people in society have used – or at least tried – tools like ChatGPT and other generative AI models. There seems to be new stories about amazing capabilities of the models every day, and there also seems to be new stories about concerns about the technology – such as impact on jobs and the tendency for generative AI models to hallucinate – every day as well.
The excitement about the potential for generative AI has extended to the governance & compliance community, and providers have been rushing to quickly integrate generative AI capabilities and leverage the capabilities of large language models (LLMs) into their products. One of the capabilities that has received considerable attention is the ability for LLMs to apply auto summarization to document collections to enable legal & compliance professionals to quickly understand information within a document collection and use it to streamline decision making regarding specific documents. In this article, we’ll discuss how generative AI auto summarization works, how it can be applied to support eDiscovery & Surveillance workflows and the benefits and challenges of using auto summarization in eDiscovery.
What is Auto Summarization?
Auto summarization in the context of large language models involves generating concise summaries of longer texts. The aim is to capture the core ideas and essential information in a much shorter form. This process can be particularly valuable for digesting large volumes of text or understanding the key points of complex documents without needing to read through the entire content.
Auto summarization…can be particularly valuable for digesting large volumes of text or understanding the key points of complex documents without needing to read through the entire content.
Irfan Shuttari, Director of eDiscovery Strategy, Product Management at Veritas.
As discussed in this detailed research paper, auto summarization provides several benefits, including:
- Summaries reduce reading/review times, and help in the selection of documents when conducting research.
- Auto summarization is free of personal biases that may be present in humans who read and summarize documents.
- Unlike humans – who get tired and whose attention span may waiver – models performing auto summarization can be more consistent in generating summaries.
The underlying techniques in auto summarization include extractive and abstractive methods:
- Extractive Summarization: This method involves selecting and compiling parts of the original text (such as sentences or phrases) to create a summary. The model identifies and extracts the most important sections directly from the text.
- Abstractive Summarization: This method involves generating new text that captures the essence of the original content but in a condensed form. It allows for more flexibility and creativity in how information is presented, as the model rephrases and synthesizes the content into a cohesive summary.
LLMs typically utilize a combination of these techniques in summarizing documents.
Given that abstractive summarization involves both a retrieval and generative component, it could be seen as a form of retrieval-augmented generation (RAG), which uses natural language processing to combines the retrieval of relevant information from a large dataset with the generation capabilities of a language model. However, RAG and abstractive summarization are typically used for different purposes. RAG is applicable to a range of tasks including but not limited to summarization, while abstractive summarization is about extracting the essence of a document into a summary.
Applying Auto Summarization to eDiscovery
As discussed in the Market Size Forecast for 2023-2028 published by ComplexDiscovery last November, the Review task commands the largest share of eDiscovery spending by far with 65 percent of total eDiscovery costs in 2023. Anyone who has conducted managed review knows that reviewers often get stuck reviewing large documents in a collection, only to often determine that the document isn’t responsive to the case. This is true even in cases with an automated review component, as some manual review is typically required to train the classification model.
As discussed in the Market Size Forecast for 2023-2028 published by ComplexDiscovery last November, the Review task commands the largest share of eDiscovery spending by far with 65 percent of total eDiscovery costs in 2023.
Irfan Shuttari, Director of eDiscovery Strategy, Product Management at Veritas.
Given the large document collections associated with eDiscovery projects in many organizations today, the potential benefits of auto summarization should be readily apparent, especially when it comes to review costs. The ability to generate case summaries of documents in the collection provides the potential to develop a much quicker understanding of each document, enabling document classifications to be performed much more quickly. As a result, many eDiscovery solutions are adding the ability to generate case summarizations – either in advance or “on the fly” – to help streamline document review and save time and costs.
Applying Auto Summarization to Surveillance
Within electronic communication surveillance, the benefit of summarization can assist review teams. Summarization of large attachments, emails, chats, or audio/video can quickly help put into context whether a specific alert requires more thorough review. Additionally, summarization can be performed against multiple items. This consolidates information from different sources into unified summaries, providing a holistic view of potential risks or compliance issues to summarize conversations across multiple channels, summarize communications for an employee or summarize communications across a group of employees over a period of time. These insights can essentially answer questions such as: “What did they discuss?” OR “What conversation were prevalent today among my team yesterday?”
With data to surveil growing larger and larger, summarizing huge volumes of communications can lead to a more efficient yet more accurate review experience, and we continue to see the surveillance market leaning towards summarization as the GenAI functional solution.
Challenges Associated with Auto Summarization
While auto summarization sounds like a great feature, like any application of generative AI technology, it’s not always accurate, which could lead to misclassifications of documents. Challenges associated with auto summarization include:
- Accuracy and Completeness: Ensuring that the summary remains faithful to the original text can be challenging. There’s a risk of altering the intended meaning or omitting critical information, especially in large documents with multiple components.
- Capturing Context and Nuance: Language is rich in nuance and context, which can be challenging for a model to fully understand and capture, especially in complex or technical texts. For example, sarcasm can present particular challenges, as can undefined acronyms. Summarization models might struggle to grasp the full context or miss subtle cues that alter the meaning, leading to summaries that might misrepresent the original text.
- Handling Diverse File Types: Files come in different types and structures, from structured documents like research papers to unstructured formats like emails, chat messages, or social media posts. Ensuring coherent and relevant summaries across different file types with different levels of text can be challenging. Some document types or even document collections may be better suited for auto classification than others.
- Adapting to Evolving Language: Language is continually evolving, with new terms, slang, and usage patterns always emerging. Keeping summarization models updated and able to understand and process these changes effectively is an ongoing challenge.
Given the challenges which can derail the quality of the output, testing and sampling of the generated summaries is recommended – just as it would be with any AI output. Taking what the model gives you at face value could lead to classification mistakes that could prove critical to your case.
Conclusion
The ability for LLMs to generate auto summarizations for documents within document collections for eDiscovery & Surveillance has the potential to be a real game changer for compliance and eDiscovery professionals. However, the key word is “potential” and best practices for validating the results of auto summarizations are still evolving. The use of LLMs to generate auto summarizations may seem like an “easy button”, but, like any other technology, should be used in a defensible manner that includes testing and validation of the results. At Veritas, we’re leaning on GenAI in establishing core functionalities for our eDiscovery & Surveillance customers while still testing the evolving technologies in the space.
Discover how Veritas Alta™ can support your eDiscovery & Surveillance needs.