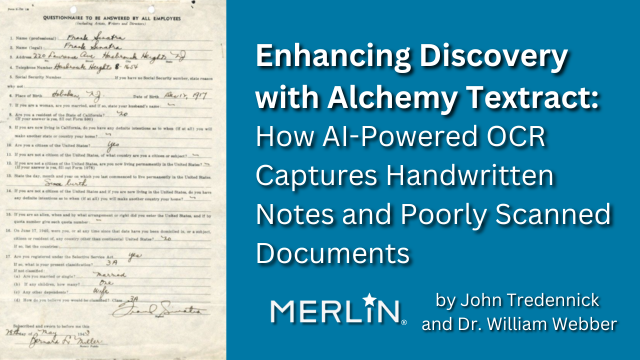
[EDRM Editor’s Note: The opinions and positions are those of John Tredennick and Dr. William Webber.]
In today’s legal landscape, investigations and discovery often involve processing thousands of complex documents. Traditional Optical Character Recognition (OCR) technology struggles with the varied document types legal professionals encounter, potentially missing crucial evidence hidden in handwritten notes, complex tables, or non-standard layouts.
This limitation was highlighted during a recent investigation in Dubai, where an international investigation firm received over 80,000 documents that had been processed using traditional OCR methods. The collection included critical investment documents, fund offering memoranda, and financial reports–many containing handwritten annotations and complex financial tables that conventional OCR had failed to capture.
The collection included critical investment documents, fund offering memoranda, and financial reports–many containing handwritten annotations and complex financial tables that conventional OCR had failed to capture.
John Tredennick and Dr. William Webber, Merlin Search Technologies.
How Alchemy Textract Works
Alchemy Textract operates differently from traditional OCR systems. Rather than simply outputting a formatted document, Textract works on a more granular level:
- It examines your document and identifies individual segments of text
- It determines the precise position of each segment on the page
- For each text element, it creates a bounding box and records both its location and content
- Our specialized transformation process then arranges these segments into proper reading order
This approach allows Textract to process documents more like a human would, recognizing both printed and handwritten text while preserving the critical relationships between elements that give documents their meaning.
Seeing the Difference: Traditional OCR vs. Alchemy Textract
Traditional OCR systems were designed for clearly printed documents with simple layouts. When confronted with the complex documents typical in legal and medical contexts, these systems often fail to capture critical information. The examples below demonstrate how Alchemy Textract outperforms traditional OCR across challenging document types.
Example 1: Handwritten Text Recognition
Handwritten notes often contain crucial information in legal investigations and medical records. Traditional OCR typically misses or misinterprets handwriting, while Alchemy Textract accurately captures these essential elements.
You can see in the example below how well Textract can read handwriting. Compare the results we received running the image through Alchemy Textract (above) with the results using a traditional ediscovery OCR process.
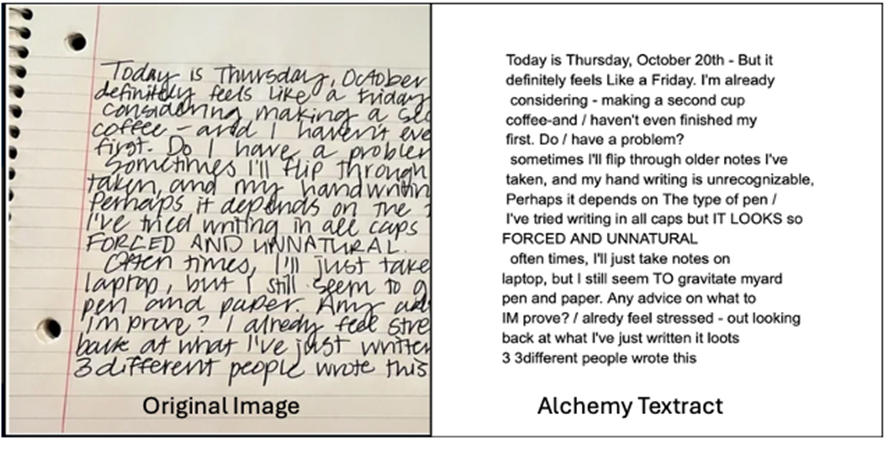
Here is the OCR retrieved from a typical ediscovery process:
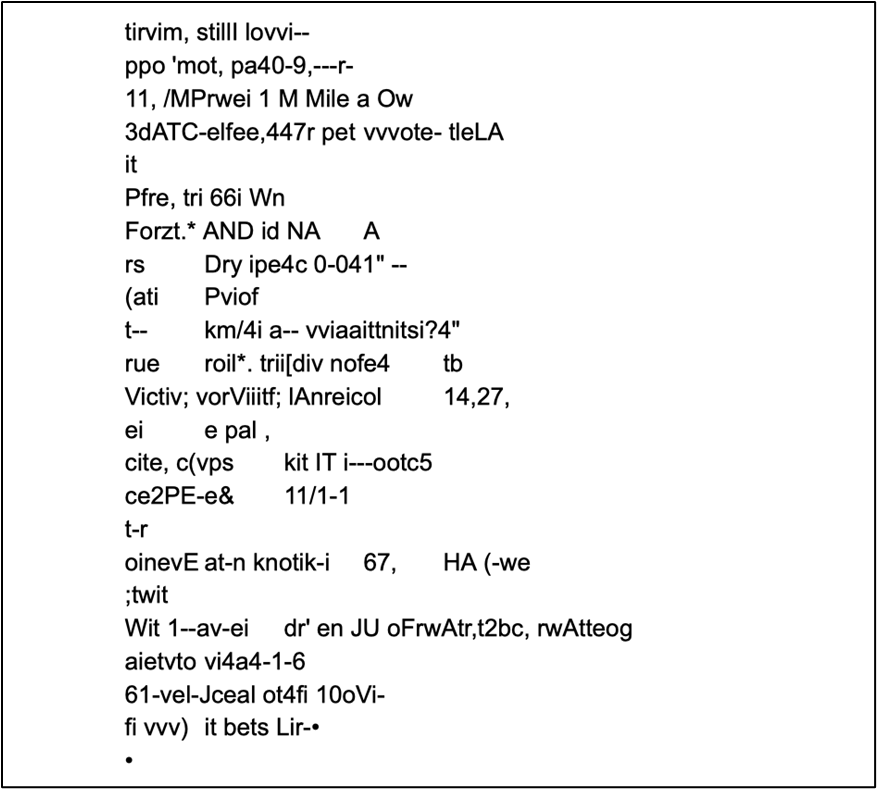
In this example:
- Traditional OCR completely failed to extract the handwritten content
- Alchemy Textract accurately captured the complete handwritten text
- The extracted content contains critical information about timing, evidence documentation, and potential insurance coverage issues
- This information would have been completely invisible to search and AI analysis with traditional OCR
Example 2: Complex Form Processing
Forms present unique challenges with their mix of printed text, checkboxes, and handwritten entries. Alchemy Textract maintains the structural relationships that give these documents meaning. Here is the original image we ran through both OCR processes:
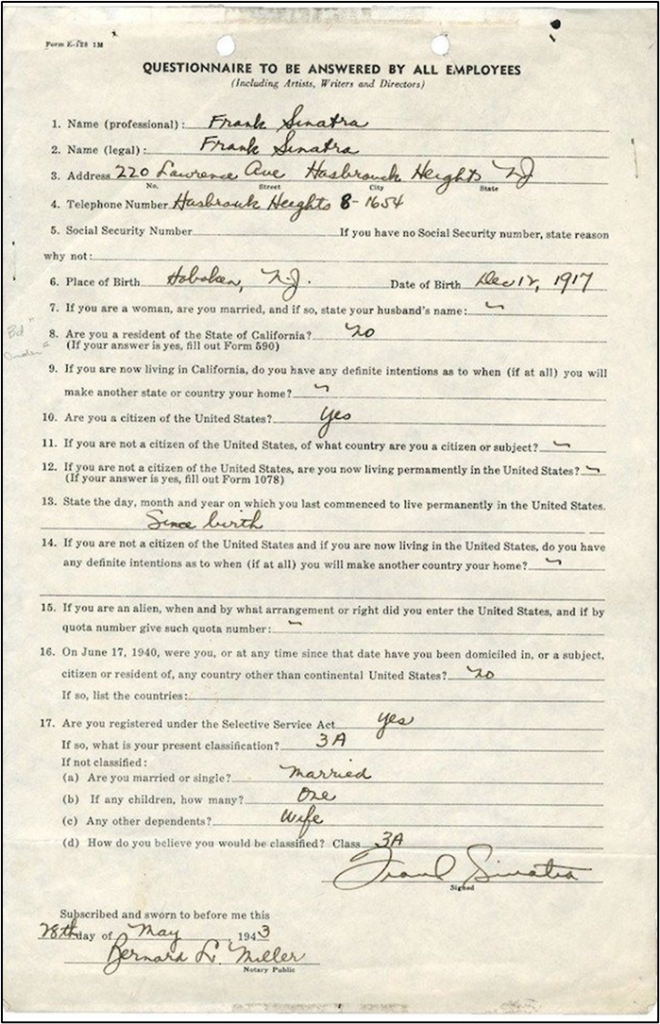
And here are the two OCR samples:
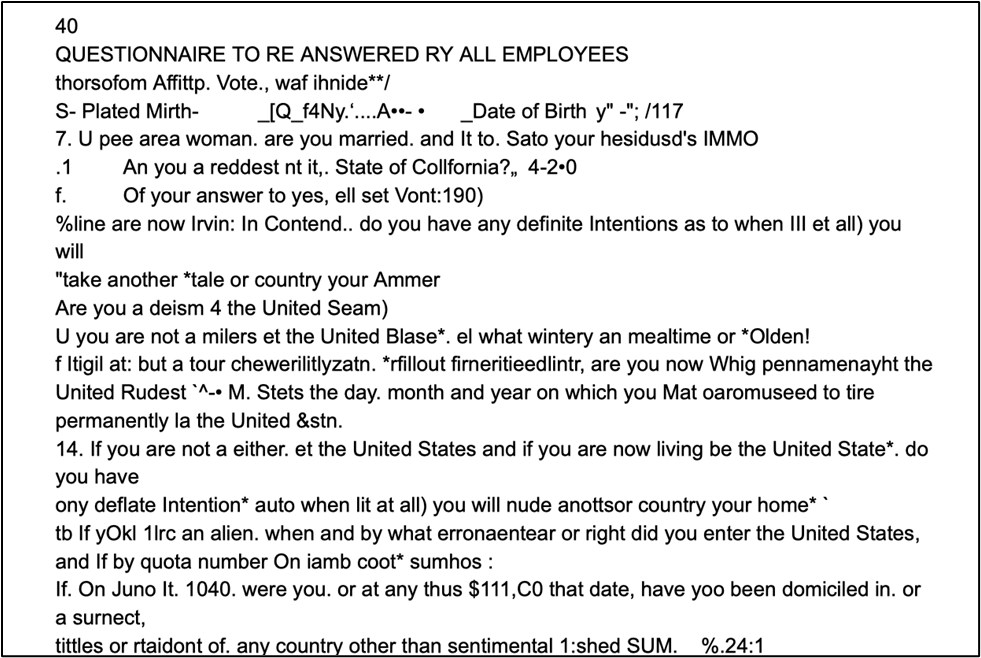
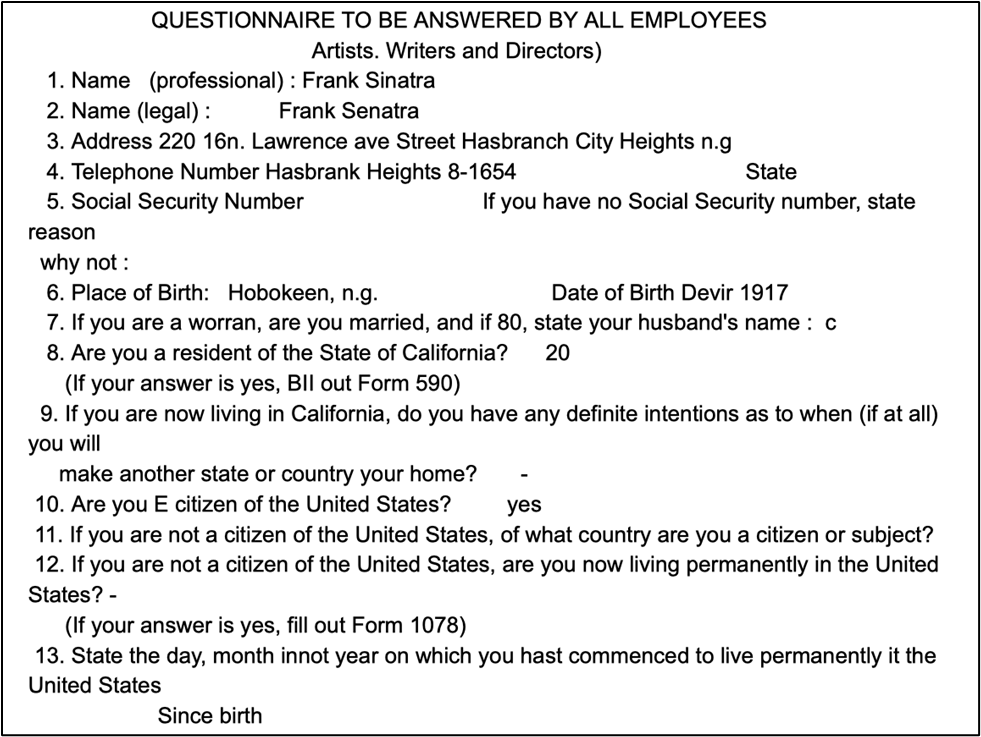
The differences are striking:
- Traditional OCR captured only a portion of the basic typed information without the critical checkbox selections or handwritten medication details
- Alchemy Textract preserved both the form structure and the handwritten entries
- The contextual relationships between fields were maintained, providing essential medical information that could be critical in litigation or patient care
These comparison examples illustrate why Alchemy Textract is essential for organizations leveraging AI for document analysis. Without accurate text extraction that preserves context and relationships, even the most sophisticated AI tools cannot deliver reliable results.
The Foundation for AI-Powered Analysis
The quality of text extraction directly impacts the effectiveness of modern discovery platforms and their AI capabilities. These systems rely on the quality of text provided to both search engines and GenAI algorithms. When text extraction is incomplete or inaccurate, even the most sophisticated AI systems cannot deliver reliable results.
With comprehensive text extraction, legal teams can:
- Find relevant documents in seconds using natural language queries
- Generate comprehensive summaries that include both typed and handwritten content
- Create detailed timelines that incorporate all document elements
- Develop fully-sourced investigation reports in minutes rather than days
The difference between traditional OCR and advanced text extraction isn’t just technical—it’s transformative for legal teams and investigators. In a recent securities investigation, handwritten margin notes captured only by advanced OCR revealed key evidence that altered the entire trajectory of the case. Law firms consistently report substantial reductions in document processing time, allowing them to focus on analysis and strategy instead of manual review.
The Future of Document Intelligence
While electronic documents dominate today’s legal landscape, complex investigations and litigation still regularly involve critical paper records, handwritten notes, and legacy documents that require OCR processing. When these situations arise, the quality of text extraction becomes a decisive factor in case outcomes.
While electronic documents dominate today’s legal landscape, complex investigations and litigation still regularly involve critical paper records, handwritten notes, and legacy documents that require OCR processing. When these situations arise, the quality of text extraction becomes a decisive factor in case outcomes.
John Tredennick and Dr. William Webber, Merlin Search Technologies.
For matters involving medical records with physician notes, regulatory filings with handwritten annotations, or complex financial documents with tables and marginalia, the ability to accurately capture all document elements often makes the difference between discovery and oversight.
Modern document intelligence requires both efficient processing of electronic files and exceptional handling of paper documents with handwritten elements. As AI continues to transform legal practice, organizations that invest in comprehensive text extraction capabilities establish the essential foundation for successful outcomes in today’s increasingly complex legal environment.
Assisted by GAI and LLM Technologies per EDRM GAI and LLM Policy.