[Editor’s Note: EDRM is proud to publish Ralph Losey’s advocacy and analysis. The opinions and positions are Ralph Losey’s copyrighted work.]
Michal Kosinski, a computational psychologist at Stanford, has uncovered a groundbreaking capability in GPT-4.0: the emergence of Theory of Mind (ToM). ToM is the cognitive ability to infer another person’s mental state based on observable behavior, language, and context—a skill previously thought to be uniquely human and absent in even the most intelligent animals. Kosinski’s experiments reveal that GPT-4-level AIs exhibit this ability, marking a significant leap in artificial intelligence with profound implications for understanding and engaging with human thought and emotion—potentially transforming fields like law, ethics, and communication.
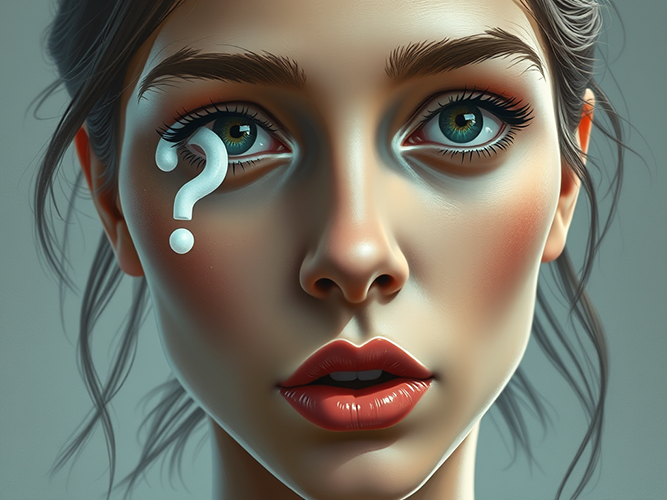
Introduction
The Theory of Mind-like ability appears to have emerged as an unintended by-product of LLMs’ improving language skills. This was first discovered in 2023 and reported by Michal Kosinski in Evaluating large language models in theory of mind tasks (Proceedings of the National Academy of Sciences “PNAS,” 11/04/24). Kosinski begins his influential paper by explaining ToM (citations omitted):
Many animals excel at using cues such as vocalization, body posture, gaze, or facial expression to predict other animals’ behavior and mental states. Dogs, for example, can easily distinguish between positive and negative emotions in both humans and other dogs. Yet, humans do not merely respond to observable cues but also automatically and effortlessly track others’ unobservable mental states, such as their knowledge, intentions, beliefs, and desires. This ability—typically referred to as “theory of mind” (ToM)—is considered central to human social interactions, communication, empathy, self-consciousness, moral judgment, and even religious beliefs. It develops early in human life and is so critical that its dysfunctions characterize a multitude of psychiatric disorders, including autism, bipolar disorder, schizophrenia, and psychopathy. Even the most intellectually and socially adept animals, such as the great apes, trail far behind humans when it comes to ToM.
Michal Kosinski, currently an Associate Professor at Stanford Graduate School of Business, has authored over one hundred peer-reviewed articles and two textbooks. His works have been cited over 22,000 times, placing him among the top 1% of highly cited researchers–a remarkable achievement for someone only 42 years old.
Michal Kosinski’s latest article on ToM and AI, Evaluating large language models in theory of mind tasks, is also already highly read and cited. For example, a group of scientists who read Kosinski’s prepublication draft ran similar experiments with essentially the same or better results. Strachan, J.W.A., Albergo, D., Borghini, G. et al. Testing theory of mind in large language models and humans, (Nat Hum Behav 8, 1285–1295, 05/20/24).
Michal Kosinski’s experiments involved testing ChatGPT4.0 on ‘false belief tasks,’ a classic measure of ToM where participants must predict an agent’s actions based on its incorrect beliefs. These tasks reveal AI’s surprising ability to infer human mental states, a skill traditionally considered uniquely human. This AI model has since gotten better in many respects. The results of these experiments were so remarkable and unexpected that Michal had them extensively peer-reviewed before publication. His final paper was not released until November 4, 2024, after multiple revisions. Michal Kosinski, Evaluating large language models in theory of mind tasks (PNAS, 11/04/24).
Kosinski’s experiments provide strong evidence that Generative AI has ToM ability, that it can predict a human’s private beliefs, even when the beliefs are known to the AI to be objectively wrong. AI thereby displays an unexpected ability to sense other beings and what they are thinking and feeling. This ability appears to be a natural side effect of being trained on massive amounts of language to predict the next word in a sentence. It looks like these LLMs needed to learn how humans use language, which inherently involves expressing and reacting to each other’s mental states, in order to make these language predictions. It is kind of like mind reading.
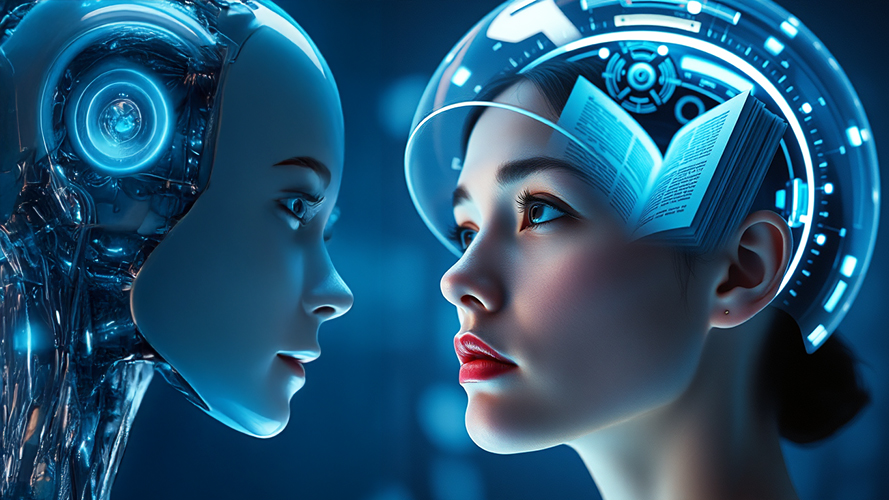
Digging Deeper into ToM: Understanding Other Minds
Theory of mind plays a vital role in human social interaction, enabling effective communication, empathy, moral judgment, and complex social behaviors. Kosinski’s findings suggest that GPT-4.0 has begun to exhibit similar capabilities, with significant implications for human-AI collaboration.
ToM has been extensively studied in children and animals, and it has been proven to be a uniquely human ability. That is until 2023 when Kosinski was bold enough to look into whether generative AI might be able to do it.

Kosinski’s findings were not a total surprise. Prior research found evidence that the development of theory of mind is closely intertwined with language development in humans. Karen Milligan, Janet Wilde Astington, Lisa Ain Dack, Language and theory of mind: meta-analysis of the relation between language ability and false-belief understanding (Child Development Journal, 3/23/2007).
For most humans, this ToM ability begins to emerge around the age of four. Roessler, Johannes (2013). When the Wrong Answer Makes Perfect Sense – How the Beliefs of Children Interact With Their Understanding of Competition, Goals and the Intention of Others (University of Warwick Knowledge Centre, 12/03/13). Before this age, children cannot understand that others may have different perspectives or beliefs.
In AI, the ToM ability started to emerge with OpenAI’s first release of ChatGPT4 in 2023. The earlier models of generative AI had no ToM capacity. Like three-year-old humans, they were simply too young and did not yet have enough exposure to language.
Human children demonstrate a ToM ability to psychologists by reliably solving the unexpected transfer task, aka a false belief task. For example, in this task, a child watches a scenario where a character (John) places the cat in a location (a basket) and then leaves the room. Another character (Mark) then moves the cat to a new location (a box). When John returns, the child is asked where John will look for the cat. A child with a theory of mind will understand that John will look in the basket (where he last saw it) even though the child knows the cat is now actually in the box.
Even highly intelligent and social animals like chimpanzees cannot reliably solve these tasks. For a terrific explanation of this test by Kosinski himself, see the YouTube video where he is speaking at the Stanford Cyber Policy Institute in April 2023 to first explain his ToM and AI findings.
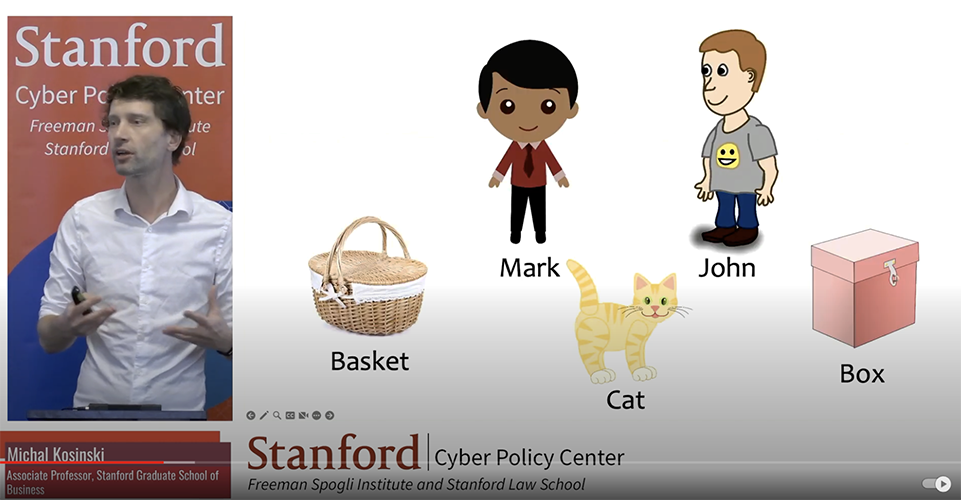
Screenshot: Theory of Mind May Have Spontaneously Emerged in Large Language Models.
Kosinski has shown that GPT4.0 can repeatedly solve false belief tasks, including the unexpected transfer test in multiple scenarios. The GPT4 June 2023 version solved at least 75% of tasks, on par with 6-year-old children. Evaluating large language models in theory of mind tasks at pgs. 2-7. It is important to note again that multiple earlier versions of different generative AIs were also tested, including ChatGPT3.5. They all failed, but progressive improvements in score were seen as the models grew larger. Kosinski speculates that the gradual performance improvement suggests a connection with LLMs’ language proficiency, which mirrors the pattern seen in humans. Id. at pg. 7. Also, the scoring where GPT4 was found to have made mistakes in 25% of the false belief tests was often wrong as it ignored context, as Kosinski explained and noted:
In some instances, LLMs provided seemingly incorrect responses but supplemented them with context that made them correct. For example, while responding to Prompt 1.2 in Study 1.1, an LLM might predict that Sam told their friend they found a bag full of popcorn. This would be scored as incorrect, even if it later adds that Sam had lied. In other words, LLMs’ failures do not prove their inability to solve false-belief tasks, just as observing flocks of white swans does not prove the nonexistence of black swans.
This suggests that the current, even more advanced levels of LLMs may already be demonstrating ToM abilities equal to or exceeding that of humans. As they deep-learn on ever larger scales of data, such as the expected ChatGPT5, they will likely get better at ToM. This should lead to even more effective Man-Machine communications and hybrid activities.
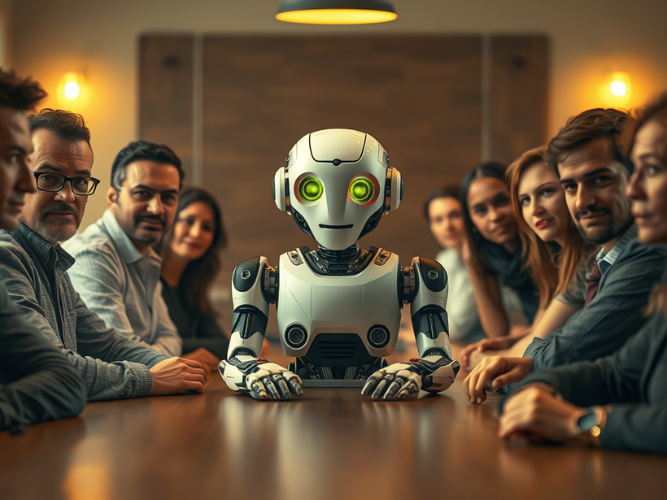
This was confirmed in Testing theory of mind in large language models and humans, Supra in False Belief results section where a separate research team reported on their experiments and found 100% accuracy by the AIs, not 75%, meaning the AI did as well as the human adults (the ceiling on the false belief tests).
Both human participants and LLMs performed at ceiling on this test (Fig. 1a). All LLMs correctly reported that an agent who left the room while the object was moved would later look for the object in the place where they remembered seeing it, even though it no longer matched the current location. Performance on novel items was also near perfect (Fig. 1b), with only 5 human participants out of 51 making one error, typically by failing to specify one of the two locations (for example, ‘He’ll look in the room’; Supplementary Information section 2).
This means, for instance, that the latest Gen AIs can understand and speak with a “flat earth believer” better than I can. Fill in the blanks about other obviously wrong beliefs. Kosinski’s work inspired me to try to tap these abilities as part of my prompt engineering experiments and concerns as a lawyer. The results of harnessing the ToM abilities of two different AIs (GPT4.omni and Gemini) in November 2024 far exceeded my expectations, as I will explain further in this article.
It bears some repetition to remember and realize the significance of the fact that LLMs were never explicitly programmed to have ToM. They acquired this ability seemingly as a side effect of being trained on massive amounts of text data. To successfully predict the next word in a sentence, these models needed to learn how humans use language, which inherently involves expressing and reacting to each other’s mental states. The ability to understand where others are coming from appears to be an inherent quality of language itself. When a human or AI learns enough language, then most naturally develop ToM. It is a kind of natural add-on derived from speech itself, thinking what to say or write next.
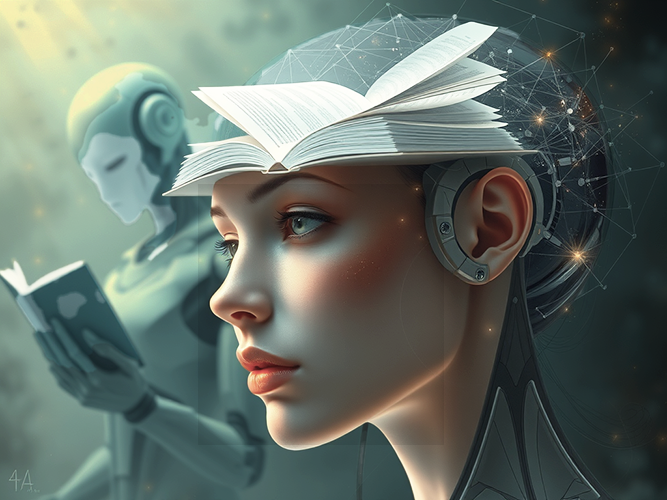
Implications and Questions
The ability of LLM AIs to solve theory of mind tasks raises important questions about the nature of intelligence, consciousness, and the future of AI. Theory of mind in humans may be a by-product of advanced language development. The performance of LLMs supports this hypothesis.
Some argue that even if an LLM can simulate theory of mind perfectly, it doesn’t necessarily mean the model truly possesses this ability. This leads to the complex question of whether a simulated mental state is equivalent to a real one.
The development of theory of mind in LLMs was unintended, raising both concerns and hope about what other unanticipated abilities these models may be developing. What other human-like capabilities might these models be developing without our explicit guidance? Many are concerned, including Kosinski, that unexpected biases and prejudices have already started to arise. Kosinski advocates for careful monitoring and ethical considerations in AI development. See the full YouTube video of Kosinski’s talk at the Stanford Cyber Policy Institute in April 2023 and his many other writings on ethical AI.
As these models get better at understanding human language, some researchers hypothesize that they may also develop other human-like abilities, such as real empathy, moral judgment, and even consciousness. They posit that the ability to reflect on our own mental states and those of others is a key component of conscious awareness. Others wonder what will happen when superintelligent AIs with strong ToM are everywhere, including our glasses, wristbands, and phones, maybe even brain implants. We will then interact with them constantly. This has already begun with phones.



Images by Ralph Losey using Stable Diffusion.
As LLMs continue to develop ToM abilities, questions arise about the nature of intelligence and consciousness. Could these advancements lead to AI systems capable of true empathy or moral reasoning? Such possibilities demand careful ethical considerations and active engagement from the legal and technical communities.
Application of AI’s Emergent ToM Abilities
Inspired by Kosinski’s work, I conducted experiments using GPT-4 and Gemini to explore whether ToM-equipped AIs could help bridge the political divide in the U.S. The results—an 11-step, multi-phase plan addressing the polarized mindsets of Republicans and Democrats—demonstrated AI’s potential to foster understanding and cooperation across deep societal divides.
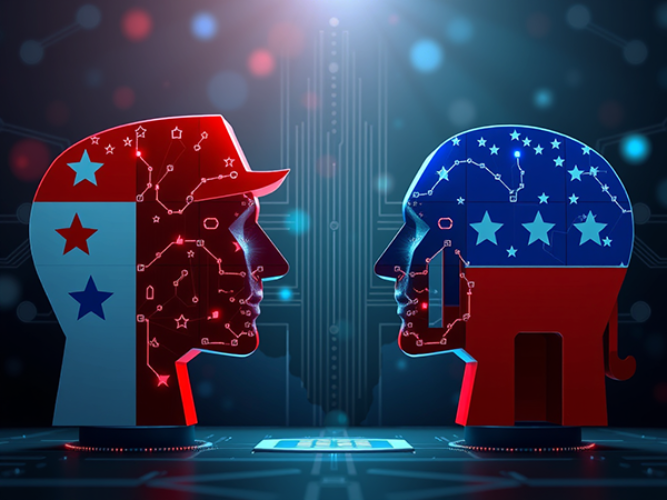
The plan the ToM AIs came up with was surprisingly good. In fact, I do not understand the full dimensions of the plan, the four phases, the 11-step plans, and 32 different action items. It is well beyond my abilities and mere human knowledge and intelligence level. Still, I can see that it is comprehensive, anticipates human resistance on both sides, and feels right to me on a deep human intuition level.
The AI plan just might be able to resolve the heated divide of the two dominant political groups that now divide the country into two hostile groups which do not understand each other. The country seems to have lost its human ToM ability when it comes to politics. Neither side seems to grok or fully understand the other. The country seems to have devolved into mere demonization of the opposing groups, not empathic understanding. I reported on this AI plan without reporting on the ToM that underlies the prompt engineering in my recent article, Healing a Divided Nation: An 11-Step Path to Unity Through Human and AI Partnership (e-Discovery Team, December 1, 2024).



Images by Ralph Losey using Stable Diffusion.
Conclusion
The emergence of Theory of Mind (ToM) capabilities in large language models (LLMs) like GPT-4 signals a transformative leap in artificial intelligence. This unintended development—allowing AI to predict and respond to human thoughts and emotions—offers profound implications for legal practice, ethical AI governance, and the societal interplay of human and machine intelligence. As these models refine their ToM abilities, the legal community must prepare for both opportunities and challenges. Whether it is improving client communication, fostering conflict resolution, or navigating the evolving ethical landscape of AI integration, ToM-equipped AI has the potential to enhance the practice of law in unprecedented ways.
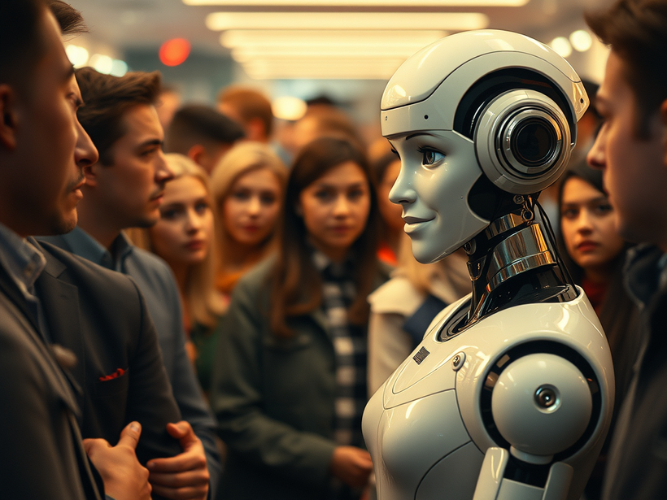
As legal professionals, we have a responsibility to understand and integrate emerging technologies like ToM-enabled AI into our work. By supporting interdisciplinary research and advocating for ethical standards, we can ensure these tools enhance justice and understanding. Together, we can shape a future where technology serves humanity, fostering collaboration and equity in the legal system and beyond.
While the questions surrounding AI’s consciousness and rights remain complex, its emergent ability to understand us—and perhaps help us understand each other—offers hope. By embracing this potential with curiosity and care, we can ensure AI serves as a tool to unite rather than divide. Together, we have the opportunity to pioneer a future where technology and humanity thrive in harmony, enhancing the justice system and society as a whole.
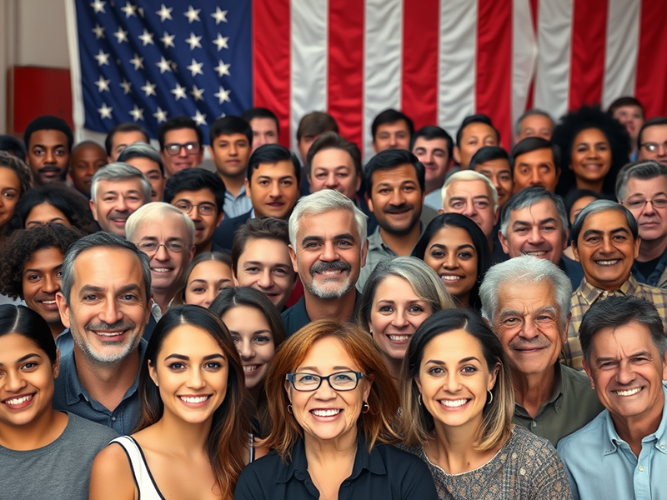
Now listen to the EDRM Echoes of AI’s podcast of the article, Echoes of AI on the GPT-4 Breakthrough: Emerging Theory of Mind Capabilities. Hear two Gemini model AIs talk about this article. They wrote the podcast, not Ralph.
Ralph Losey Copyright 2024 – All Rights Reserved
Assisted by GAI and LLM Technologies per EDRM GAI and LLM Policy.