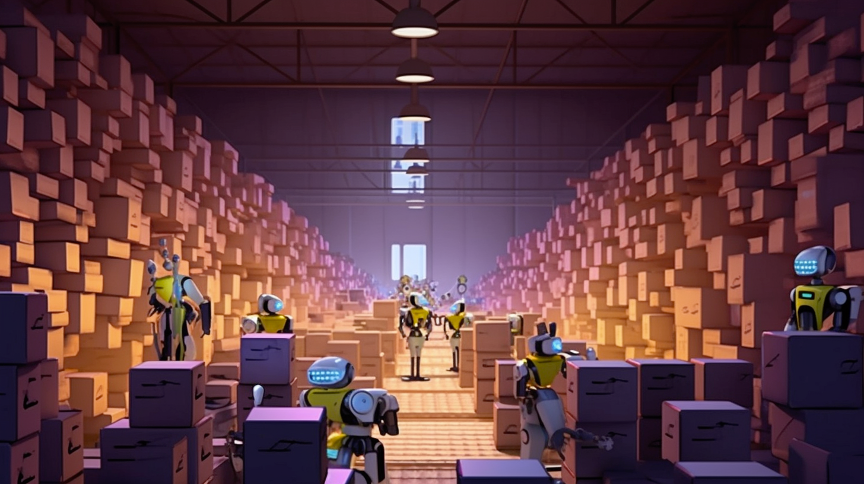
By John Tredennick and Dr. William Webber
{Editor’s Note: This article first appeared in the May 24, 2023 issue of LegalTech News from Law.com, a publication of American Lawyer Media.]
Since its release in late 2022, ChatGPT has captivated the world, drawing a million users in its first five days and totaling over 100 million today. Whether you are fearful or excited, there is little doubt we have entered a transformational era, much like the invention of the printing press, the steam engine, cell phones, and the Internet itself. The extent and speed of ChatGPT’s progress (and of competitor systems) remains the only open question.
Like everyone else, we have read lots of flowery articles about the potential of ChatGPT to redefine the professional world. In this article, we intend to go beyond generalities by demonstrating five specific ways legal professionals can use ChatGPT (and its underlying GPT engine) to make ediscovery more efficient and cost-effective.
Using GPT and a basic search engine, we can show how legal professionals will soon take advantage of Large Language Models like GPT to streamline their ediscovery processes and better understand the key documents in their cases.
Prepare to be amazed.
Our Topic for This Exercise
Most ediscovery efforts start with a Rule 34 Request for Production. For this exercise, we will use one of the legal track topics from NIST’s annual Text Retrieval Conference (TREC), which was created in part to test the ability of different machine learning algorithms to find relevant documents:
“2000 Recount — All documents concerning the contested result of the 2000 presidential election.”
Our discovery collection consists of approximately 290,000 emails that Jeb Bush made public from his two terms as Governor of Florida. TREC used these emails for its programs and they provide a good test bed for the integration of GPT and traditional ediscovery search and review methods. Our GPT system makes these documents available for interactive analysis and interrogation through GPT.
1. Using GPT to Help with Keyword Searches
One of the first steps in addressing an RFP is to identify appropriate keywords and build a Boolean search. While we can lift terms from the topic request, experienced ediscovery professionals know that the process can require hours of time testing keywords and analyzing search results to come up with a comprehensive list of terms to include in the search.
Let’s start by asking GPT to help us determine keywords for this topic with the following prompt (a question or instruction sent to GPT). Watch as we have a conversation with GPT to flesh out our keyword list and turn it into a Boolean search:
Prompt: Here is my topic: “2000 Recount — All documents concerning the contested result of the 2000 presidential election.” Can you suggest keywords to help make my search better? GPT: Keywords that could improve the search for “2000 Recount — All documents concerning the contested result of the 2000 presidential election” include: election manipulation, voting machines, Diebold, security, paper trail, ballot review, consortium, statewide hand count, overvoted ballots, polls, voting, election, fraud, revote, federal law, media projection, electoral college, standard ballot, personnel, troubleshooting, felony list, and legitimate voters. These keywords are supported by the following documents: – Document 018716: Mentions “election manipulation,” “voting machines,” “Diebold,” “security,” and “paper trail.” – Document 099018: Mentions “ballot review,” “consortium,” “statewide hand count,” “overvoted ballots,” and “outcome.” – Document 064808: Mentions “polls,” “voting,” “election,” “contested result,” “legal maneuvers,” “ambiguous conclusions,” “procedures,” “previous elections,” and “precedent.” Document 124142: Mentions “contested result,” “fraud,” and “revote.” – Document 065969: Mentions “federal law,” “media projection,” “polls,” “electoral college,” “standard ballot,” “voting machine,” “recount,” and “Florida votes.”- Document 123697: Mentions “voting equipment,” “personnel,” “troubleshooting,” “felony list,” “legitimate voters,” and “right to vote.” – Document 145857: Mentions “touch screen voting machines,” “security,” “open election records,” “Perfect Voting System,” “investigation,” “Georgia,” “Maryland,” “audit,” and “public inspection.” – Document 123823: Mentions “recount,” “contested result,” and “AP Election Documentary.” – Document 064796: Mentions “recount,” “ballots,” “Secretary of State,” and “deadline.” – Document 113386: Mentions “recount,” “contested result,” and “political careers.” – Document 113389: Mentions “recount,” “contested result,” “political career,” and “Katherine Harris.” Prompt: Can you suggest any other keywords I might use to improve my search? GPT: Here are some additional keywords that could improve your search: – Hanging chads Butterfly ballot Supreme Court Gore v. Bush Florida Supreme Court Bush v. Palm Beach County Canvassing Board Bush v. Gore Absentee ballots Voter disenfranchisement Voter suppression Partisan politics Legal challenges Election reform Public opinion Media coverage Political polarization These keywords are supported by the documents mentioned earlier, as well as other documents related to the 2000 presidential election recount. Prompt: Thanks. Can you build me a Boolean search based on all of the keywords you have suggested? GPT: Certainly! Here’s a Boolean search string that includes all of the keywords suggested: (“election manipulation” OR “voting machines” OR “Diebold” OR “security” OR “paper trail” OR “ballot review” OR “consortium” OR “statewide hand count” OR “over voted ballots” OR “polls” OR “voting” OR “election” OR “fraud” OR “revote” OR “federal law” OR “media projection” OR “electoral college” OR “standard ballot” OR “touch screen voting machines” OR “open election records” OR “Perfect Voting System” OR “investigation” OR “Georgia” OR “Maryland” OR “audit” OR “public inspection” OR “hanging chads” OR “butterfly ballot” OR “Supreme Court” OR “Gore v. Bush” OR “Florida Supreme Court” OR “Bush v. Palm Beach County Canvassing Board” OR “Bush v. Gore” OR “absentee ballots” OR “voter disenfranchisement” OR “voter suppression” OR “partisan politics” OR “legal challenges” OR “election reform” OR “public opinion” OR “media coverage” OR “political polarization”) You can use this search string in combination with your preferred search engine or database to find relevant documents related to the 2000 presidential election recount. |
Read the original article chronicling the entire experiment, including using GPT to summarize documents, using GPT to answer questions, using GPT to create an investigation report, and using GPT for document review.