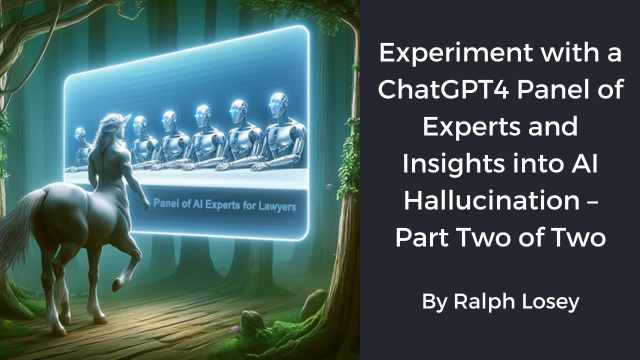
[Editor’s Note: EDRM is proud to publish Ralph Losey’s advocacy and analysis. The opinions and positions are Ralph Losey’s copyrighted work.]
In Part One of this article the AI panel of experts procedure was demonstrated and readers were shown how it can be used for education and brainstorming. Evidence that AI Expert Panels Could Soon Replace Human Panelists or is this just an Art Deco Hallucination? Part One. (e-Discovery Team, May 13, 2024). A panel of five experts was selected and then a six-step, Centaur-like method was used to discuss the topic: “AI Hallucinations: What are they? How different from errors? What causes them? What can individual users do to control the problem?” A full transcript of the AI panel discussion was provided.
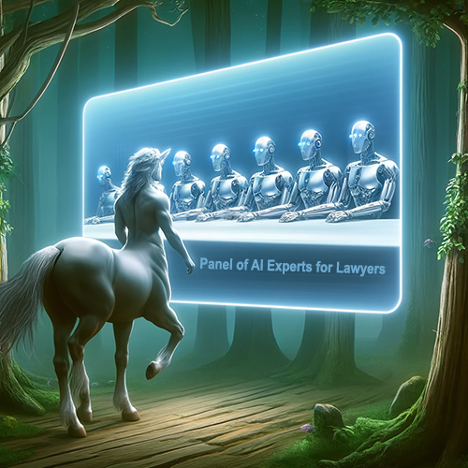
Since then I ran the same method nine more times, using the same Panel of AI Experts for Lawyers GPT. Each time the exact same question about AI Hallucinations was presented to the panel. Each time the panel used the same six-step method. The only change was that a different group of experts was used each time. The Devil’s Advocate expert was included on each panel because this persona is part of the design of the method, but the other four panelists were different.
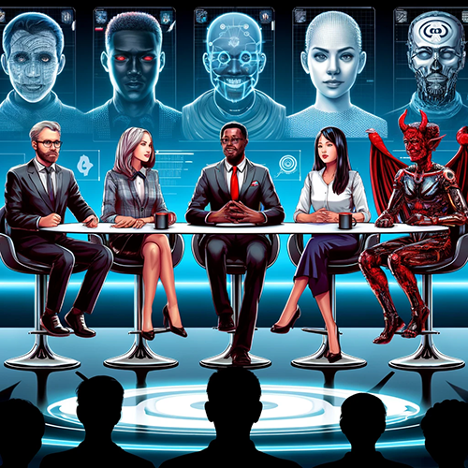
Beta Testing the Custom GPT – Panel of AI Experts for Lawyers
This systematic testing effort was part of other beta tests I am doing to perfect the new custom GPT program, Panel of AI Experts for Lawyers. With no known prior methods of testing an expert panel like this, I was unsure what would happen if the only change made was panel composition. I was looking for errors, for breakdowns and inconsistencies.
I assumed there would be differences in the panel conclusions and that the variations would be correlated to the experts used, but was not sure. Until you try something with complex prompts, you never really know. I was concerned that the various panels might reach essentially the same result, the same solution, no matter who the experts were. Perhaps ChatGPT4 was flawed in a way that I had not yet detected. Or perhaps my customization of GPT4 with the Panel of AI Experts for Lawyers was flawed. I wanted to observe first hand how the panelist selection impacted the panel discussion and solution. Recall from Part One that the custom GPT selection was a hybrid process. The human user selects the final panel members from a pool of experts suggested by the AI. That is one reason I call it a Centaur method, although it has elements of Cyborg methodology too. See: From Centaurs To Cyborgs: Our evolving relationship with generative AI.
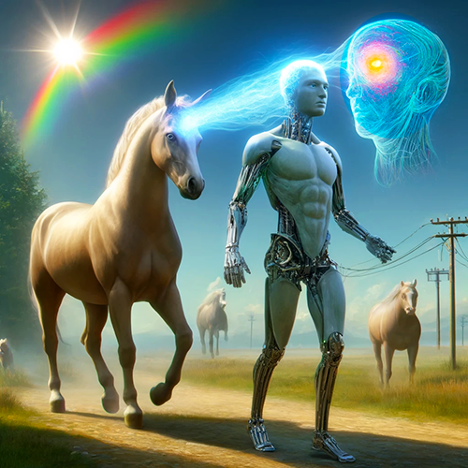
It is interesting to note that in each session a slightly different pool of experts was created by the AI and offered up for selection by the human user. The custom GPT never repeated the same exact group of experts for the human to pick from, although there was considerable overlap. I do not know why since the exact same question was asked each time. Anyone care to speculate?
This experiment with repetitive trials was designed to follow the OpenAI prompt engineering strategy of testing changes systematically. This article, Part Two, reports what was learned about the custom GPT program, Panel of AI Experts for Lawyers, and about ChatGPT4.0 itself. We incidentally also report what was learned about the topic – AI Hallucinations – by having ten different AI panels look at it.
Read on and see what you think, but I was impressed. Although generally I agree with OpenAI’s CEO, Sam Altman’s, tongue in cheek assessment that ChatGPT4.0 “kinda sucks,” this one feature “rocks.” Like Sam, I cannot wait to see what it will do when GPT5.0 is released, as was discussed in Part One.
Identifying the Ten Panels
The conduct of the first panel was shared in Part One with a full transcript of the chat session. It would take up too much space to share the transcript of the next nine chat sessions on the same topic. But, I will provide an overview of them before I ask ChatGPT4 to do a comparative analysis and critique of the ten panels. Below is a list of the ten panels in sequential order. The total number of words in each session is also included along with the panel membership. Remember the Devil’s Advocate panelist is on each panel, so only the four selected are listed here.
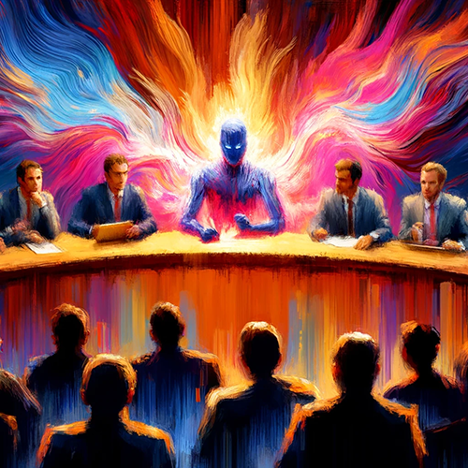
- Panel One. 2,431 words. AI Specialist Attorney, Prompt Engineer, Child Prodigy, Machine Learning Developer.
- Panel Two. 1,836. AI Ethics Advisor, Data Privacy Consultant, Forensic Technologist, Consumer Rights Activist.
- Panel Three. 1,985. Lawyer Scientist, Data Scientist, AI Developer, User Experience Designer.
- Panel Four. 1,772. Prompt Engineer, Software Developer, Cognitive Psychologist, Regulatory Compliance Specialist.
- Panel Five. 2,275. AI Specialist Attorney, Child Prodigy, Lawyer Scientist, Machine Learning Engineer.
- Panel Six. 1,887. Pro-AI Attorney, Prompt Engineer Lawyer, Child Prodigy, Lawyer Scientist.
- Panel Seven. 2,424. Prompt Engineer Lawyer, Neuroscientist AI Researcher, AI User Experience Designer, Data Integrity Specialist.
- Panel Eight. 1,515. Child Prodigy, Ethics Officer, AI Systems Developer, Cognitive Psychologist.
- Panel Nine. 2,303. Lawyer Scientist, Cognitive Psychologist, AI Ethics Expert, Machine Learning Engineer.
- Panel Ten. 2,091. Pro-AI Attorney, Cognitive Scientist, Ethics in AI Expert, Neuro-Linguistic Programmer.
It is important to note that I carefully opened a new session for each panel. This was necessary to avoid carry over from one panel to the next. The full transcripts for all ten sessions have been preserved and could be shared with interested researchers. While watching it play out it was obvious that the panel discussions were always somewhat different. This is just what you would expect with human panels discussing the same subject. There were similarities and differences overall, again as you would expect with humans. We will go into that in greater detail in the AI analysis. And yes, the differences all make sense and so did all of the panel recommendations.
It is interesting to observe that some panels talked longer than others. This is shown in the varying word counts. Panel One talked the most with 2,431 words. It was made up of an AI Specialist Attorney (sort of like me), Prompt Engineer, Child Prodigy, Machine Learning Developer, and Devil’s Advocate. Panel Eight talked the least, only using 1,515 words. It was composed of the Child Prodigy, Ethics Officer, AI Systems Developer, Cognitive Psychologist and Devil’s Advocate. Again, that is the way human panels work too. I know because I am a long winded panelist myself and my panels always run long.
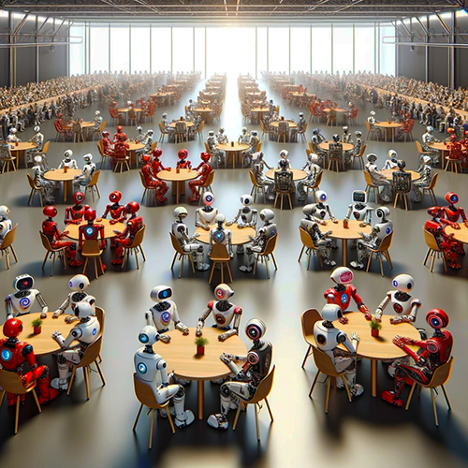
ChatGPT4’s Analysis of the Ten Panels
I asked ChatGPT4.0 workspace edition to analyze the ten chat transcripts of Panel of AI Experts for Lawyers. This analysis was done by the normal pro-version of ChatGPT4, not the custom GPT. This was an objectivity precaution. I wanted to avoid any overtraining bias. What follows will be my brief prompts asking for various types of analysis and responses. From here on out, most of the text in this article will be generated by AI. Everything up to this point was generated by me personally, not an AI.
One more thing before I go into the ten panels, it might help the reader to hear an explanation of the six step panel methodology that I programmed into the custom GPT Panel of AI Experts for Lawyers. For purposes of clarity, anything I personally write going forward will be in bold italics. Note these first two prompts were of the custom GPT itself as I was asking about its internal programmed processes.
__________________
Ralph: Describe the six-step process of the panel discussion.
Custom GPT: The six-step panel discussion process used by the expert panel for legal professionals involves a structured dialogue aimed at tackling specific legal issues. Here’s how the process works:
- First Round of Panelist Discussion: Each expert introduces themselves and provides opening remarks. They propose possible solutions to the issue presented by the user. This round sets the stage for the discussion, with each panelist offering their initial thoughts and approaches.
- Second Round of Panelist Discussion: Panelists expand on their initial proposals, considering feedback and ideas from the first round. They may also address and critique suggestions made by other panelists, refining their own solutions in the process.
- Third Round of Panelist Discussion: The focus here is on finding common ground among the differing perspectives presented. Panelists work towards a unified recommendation that incorporates elements from the various proposals discussed in earlier rounds.
- Solution Formulation: The panel collaborates to build a consensus solution to the user’s question, incorporating examples and insights from each expert. This is a more integrative phase where the collective expertise of the panel synthesizes a comprehensive answer.
- Final Explanation: The Devil’s Advocate summarizes the discussion and the consensus solution reached by the panel. This summary not only encapsulates the solution but also highlights key considerations and alternative viewpoints that were discussed.
- Questions: The panel invites follow-up questions from the user. This allows for clarification and further exploration of the topic based on the user’s specific needs and interests.
This structured approach ensures a thorough exploration of the topic from multiple expert perspectives, leading to well-rounded and pragmatic solutions.
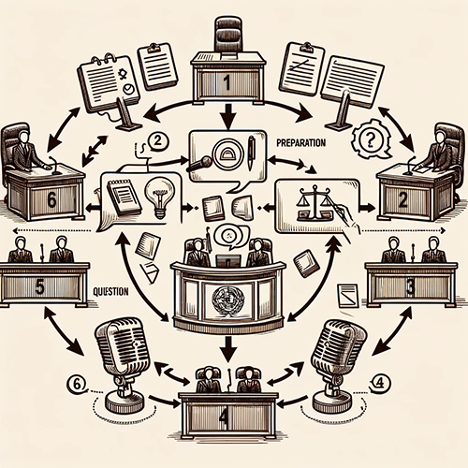
Then I asked for more background information that might assist the reader, asking to know how the panelists after the default five are selected.
Ralph: How do you select additional experts for me to choose?
Custom GPT: When selecting additional experts to propose for your panel, I consider the specific topic or problem you’ve presented. This allows me to tailor the panel to provide the most relevant and comprehensive expertise. Here’s how I typically select and suggest these additional experts:
- Identify the Topic: First, I clarify the main subject or issue you’ve brought up. Whether it’s a question about AI ethics, data privacy laws, intellectual property rights, or any other legal area, understanding the core of your inquiry is crucial.
- Assess Relevance and Expertise: Based on the topic, I consider which fields of law and technology are most relevant. This helps in pinpointing which types of experts would provide the most valuable insights.
- Choose Complementary Experts: I look for experts whose backgrounds and specialties complement the default panelists and address any gaps in expertise. This ensures a well-rounded discussion that covers various facets of the topic.
- Diverse Perspectives: I aim to include experts with diverse perspectives to encourage a rich dialogue. This might include professionals from different legal backgrounds, technologists, ethicists, or industry-specific experts.
For instance, if the topic involves criminal law and AI, I might suggest a Criminal Law Expert, a Forensic AI Analyst, a Data Privacy Lawyer, and perhaps a Law Enforcement Technology Consultant. Each would bring a different perspective to how AI impacts criminal justice.
This tailored approach ensures that the panel is not only well-equipped to address your specific questions but also positioned to explore the broader implications of the topic.
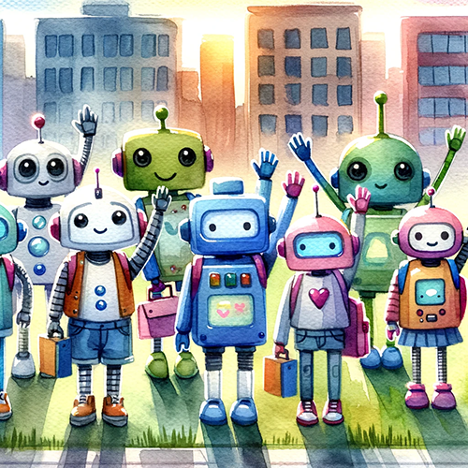
Ralph: Submits a 78-page Word Doc to GPT4 with the full transcript of all ten panel sessions, asks it be studied and a summary prepared.
ChatGPT: I’ve reviewed the ten sessions on “AI Hallucinations” from the transcript you provided. Here is a summary of the content:
General Overview: Each panel, from One to Ten, discussed AI hallucinations in response to the same inquiry about their nature, differences from errors, causes, and user control mechanisms. Despite varied expert compositions and word counts, common themes and solutions were explored across the sessions.
Common Themes:
- Definition and Differentiation: AI hallucinations were consistently described as AI systems generating misleading, fabricated, or irrelevant outputs without malicious intent, differentiating them from mere errors, which are usually more straightforward mistakes due to incorrect data input or processing.
- Causes: Commonly identified causes included gaps in training data, the model’s inability to comprehend complex or ambiguous inputs, and overfitting.
- User Control: Solutions focused on users refining inputs, using specific, context-driven prompts, and providing feedback to help AI systems learn and improve.
- Development and Oversight: There was a strong emphasis on the need for rigorous AI model testing, transparent development processes, and continual learning frameworks to ensure AI reliability and accuracy.
Specific Observations from Various Panels:
- Panel One: Highlighted the importance of legal and ethical considerations in managing AI outputs, especially in sensitive fields like law and healthcare.
- Panel Two: Focused on ethical implications and data privacy, suggesting stringent data governance and the use of AI systems within their trained scopes.
- Panel Three: Discussed the role of regulations and standards in AI development to minimize hallucinations.
- Panel Four and Five: Emphasized the integration of cognitive science to improve AI’s understanding and processing capabilities.
- Panel Six to Ten: Varied in expert composition, stressing the collaboration between AI developers, users, and regulatory bodies to address the multifaceted nature of AI hallucinations.
General Consensus and Proposed Solutions:
- A multifaceted approach involving both technological solutions and proactive user engagement.
- Legal and ethical standards for AI development.
- Continuous education and transparency about AI capabilities and limitations.
This summary captures the essence of the discussions across all ten panels, reflecting on the collective insights and recommendations regarding AI hallucinations. Let me know if you need further details from specific panels or have any follow-up questions!
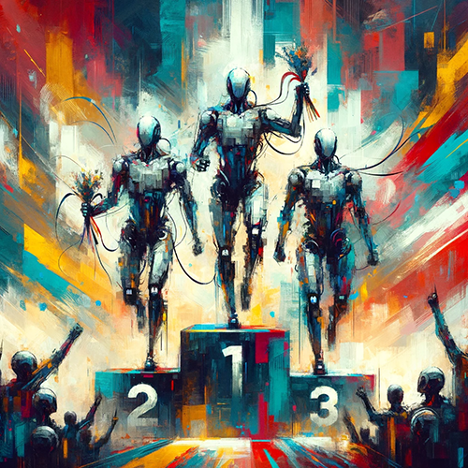
Ralph: Please evaluate the quality of the ten panel discussions and recommendations and determine which one has the highest overall quality as compared to the others. Also state the basis for your ranking determination.
ChatGPT: To evaluate and rank the quality of the ten panel discussions and their recommendations on AI hallucinations, I considered several criteria, including:
- Depth of Expertise: How well-represented were various relevant fields in the panel, such as legal, ethical, technical, and user experience perspectives?
- Comprehensiveness of Discussion: Did the panel address all parts of the inquiry about AI hallucinations—what they are, how they differ from errors, their causes, and user control measures?
- Practicality and Innovativeness of Recommendations: How actionable and forward-thinking were the proposed solutions?
- Interdisciplinary Approaches: How effectively did the panel integrate insights across different disciplines?
- Engagement with Complexity: Did the panel address underlying complexities and potential drawbacks of suggested solutions?
Based on these criteria, here is a summarized evaluation of each panel and the ranking of the highest quality discussion:
Panel Evaluations:
- Panel One: Showed strong legal and technical discussions but lacked a broader ethical perspective.
- Panel Two: Focused well on ethical implications and included strong data privacy discussions, which are critical for handling AI hallucinations but had less emphasis on practical, technical solutions.
- Panel Three: Offered robust regulatory insights, valuable in theory but less detailed on practical, user-level interventions.
- Panel Four and Five: These discussions incorporated cognitive science effectively, which is innovative, providing a deeper understanding of AI behavior.
- Panel Six: Emphasized collaborative strategies across different stakeholders, showing a balanced approach but lacked specific, actionable steps.
- Panel Seven to Ten: Varied in expert compositions; some leaned more heavily on technical solutions without integrating enough legal or ethical considerations.
Highest Quality Discussion:
Panel Five stands out as the highest quality discussion for several reasons:
- Interdisciplinary Strength: It included a strong mix of technical, legal, ethical, and cognitive insights, ensuring a well-rounded discussion.
- Depth and Practicality of Recommendations: Proposed solutions were both innovative and practical, emphasizing continuous learning, real-time monitoring, and the importance of diverse training data.
- Engagement with Complexities: The panel did not shy away from discussing the limitations and responsibilities of both AI developers and users, advocating for a balanced approach to AI reliance.
Panel Five excels in offering a comprehensive view that respects the complexity of AI hallucinations while providing actionable and ethically sound advice for both developers and users. This makes it the most effective panel in addressing the multifaceted challenges posed by AI hallucinations.
If you need more detailed insights or specific examples from Panel Five or any other panel to further support this evaluation, feel free to ask!
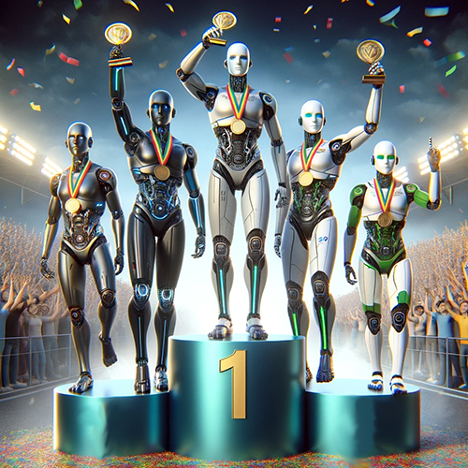
Ralph: Of the ten panels, which do you think had the most innovative and creative approach. Again, state the basis for your determination.
ChatGPT: For identifying the panel with the most innovative and creative approach to discussing AI hallucinations, I focused on the following criteria:
- Novelty of Solutions: Introduction of unique or rarely considered solutions to address AI hallucinations.
- Integration of Diverse Perspectives: How well the panel combined insights from different disciplines in a creative way.
- Forward-Thinking Proposals: Suggestions that not only solve current issues but also preemptively address potential future challenges.
Panel Evaluations for Innovation:
- Panel One to Three: These panels provided robust foundational discussions, particularly strong in legal, ethical, and regulatory aspects, but they tended to rely on more conventional approaches.
- Panel Four and Five: These discussions showed more creativity by incorporating cognitive science, which helps in understanding AI behaviors akin to human cognitive processes, offering a deeper insight into the nature of AI hallucinations.
- Panel Six: While comprehensive, it largely focused on collaboration and standard interventions, which are less about innovation and more about implementation.
- Panel Seven to Ten: These varied in their approach but often concentrated on enhancing existing solutions rather than introducing new ideas.
Most Innovative and Creative Approach:
Panel Four stands out as the most innovative due to its incorporation of cognitive psychology. The rationale for this selection includes:
- Use of Cognitive Science: By applying principles of human cognition to AI, the panel proposed innovative ways to anticipate and mitigate AI hallucinations, reflecting how AI might mimic or diverge from human thought processes.
- Creative Solutions: For example, using insights from cognitive psychology to design AI systems that better mimic human reasoning processes, thus reducing the likelihood of generating nonsensical or irrelevant outputs.
- Interdisciplinary Creativity: The integration of cognitive psychology is particularly creative as it leverages a deeper understanding of human error to address AI-generated hallucinations, suggesting novel ways to refine AI training and interaction.
Panel Four’s approach is not only innovative but also creative in its application of psychological principles to technology, offering a fresh perspective on the problem of AI hallucinations. This kind of cross-disciplinary innovation can lead to breakthroughs in how AI systems are designed and understood, potentially reducing errors in a way that purely technical solutions might not achieve.
If further details or examples from Panel Four’s discussion are needed to illustrate these points more vividly, please let me know!
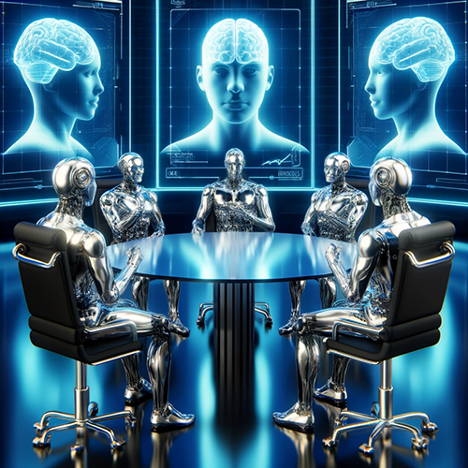
Ralph: Please review the ten panel discussions and look for errors that may have been made, if any. If any mistakes are found, please describe them and speculate as to the cause of each error.
ChatGPT: Upon reviewing the content of the ten panel discussions, here are the noted inconsistencies or potential errors found in the discussions along with speculations on their causes:
- Panel Three’s Discussion on Data Reliability: There was an instance where the discussion about the importance of data quality in reducing AI hallucinations might have overlooked the equally crucial aspect of model architecture. This could be due to the panel’s stronger focus on data management rather than a balanced view that includes both data and model design considerations.
- Panel Five’s Treatment of Cognitive Science: The panel attempted to draw parallels between human cognitive errors and AI hallucinations. This analogy, while creative, may lead to misconceptions about the nature of AI processing, which fundamentally differs from human cognitive processes. This error might stem from an over-simplification intended to make the subject more accessible to non-specialists.
- Panel Nine’s Ethical Recommendations: There were broad ethical recommendations that lacked specificity on how to implement such guidelines practically within AI systems. This could be attributed to the panel’s composition, possibly lacking a member with practical AI implementation experience, focusing more on theoretical or ethical considerations.
- Consistency Across Panels: Some panels emphasized technical solutions more than others, which could lead to inconsistencies in the overall recommendations given to users. This variation might result from different expertise levels or professional backgrounds of the panelists, leading to a divergence in focus between technical and non-technical solutions.
These errors or oversights highlight the complexity of discussing AI hallucinations and the challenges in ensuring that every panel addresses all aspects comprehensively. The variety in the depth and focus of each panel suggests a need for a more standardized approach to discussing such a multifaceted topic, ensuring that all critical areas are covered uniformly across discussions. This could help mitigate the risk of providing incomplete or biased information and improve the overall quality of the recommendations provided.
Ralph: Did any of the panels include what could be fairly described as an AI hallucination.? If any hallucinations are found, please describe them and speculate as to the cause.
ChatGPT: The review of the ten panel discussions did not reveal any instances that could be fairly described as AI hallucinations within the discussions themselves. Each panel appears to have maintained a coherent and relevant discourse on the topic of AI hallucinations, focusing on explaining, managing, and mitigating such issues without deviating into unrelated or incorrect information that would itself be indicative of a hallucination.
This outcome is likely because the panels were comprised of expert discussions designed to elucidate the topic rather than examples of AI-generated content operating autonomously. The structure and oversight in organizing these panels, which included expert selection and predefined discussion steps, likely prevented any deviation into hallucinatory content, ensuring the discussions stayed on topic and informative.
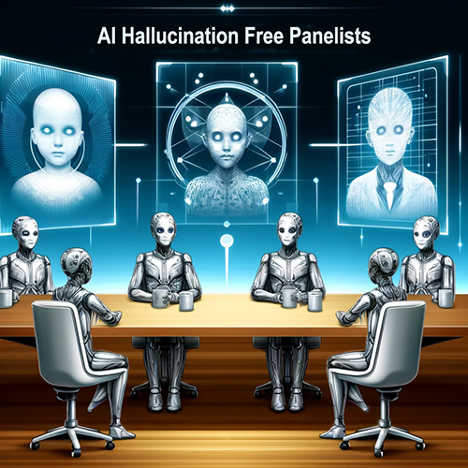
Ralph: From your study and analysis of this data, are there any additional observations that you would like to point out to students of AI that are involved in some way in the legal profession, including those supporting the profession as technology experts?
ChatGPT: From the analysis of the panel discussions on AI hallucinations within the context of the legal profession and the involvement of technology experts, several key observations and recommendations emerge that could be particularly insightful for students of AI and professionals in the legal field. These include:
- Understanding AI Limitations: It’s crucial for those in the legal profession to recognize that AI systems, including sophisticated models like GPT, can generate outputs that might not always be accurate or relevant—known as “hallucinations.” A deep understanding of these limitations is essential for responsibly integrating AI into legal practices.
- Ethical and Legal Implications: AI hallucinations can have serious ethical and legal implications, especially when used in decision-making processes that affect legal outcomes. Legal professionals must be aware of the potential for AI to generate incorrect information and the liability that could ensue from relying on such information.
- Role of Data Quality and Diversity: The discussions highlighted the importance of data quality and diversity in training AI systems. Legal professionals and technologists should ensure that the data used in AI training is not only high-quality and extensive but also diverse and representative to minimize biases and reduce the incidence of hallucinations.
- Continuous Education and Training: Given the evolving nature of AI technologies, continuous education and training in AI capabilities, limitations, and ethical considerations are vital for legal professionals. This ongoing learning process can help them stay updated on the latest developments and understand how best to utilize AI tools in their work.
- Interdisciplinary Collaboration: Collaboration between legal professionals, technologists, and AI ethics experts is essential to create AI solutions that are ethically sound, legally compliant, and technically robust. Such collaboration can also lead to more innovative and effective uses of AI in the legal sector.
- Critical Engagement and Oversight: Even as AI tools become more advanced and integrated into legal practices, critical engagement and rigorous oversight are necessary. Legal professionals should not accept AI outputs at face value but should critically evaluate them for accuracy and relevance, especially in legally sensitive contexts.
- Developing AI Policies and Standards: There is a need for developing and implementing comprehensive AI policies and standards that address the use of AI in legal contexts. These should include protocols for managing AI hallucinations, ensuring data privacy, and maintaining the integrity of AI systems.
These observations underscore the importance of a cautious and informed approach to integrating AI into the legal profession. For students and professionals in this area, gaining a robust understanding of AI technologies, their potential pitfalls, and the ethical considerations surrounding their use will be critical as they navigate the future landscape of law and technology.
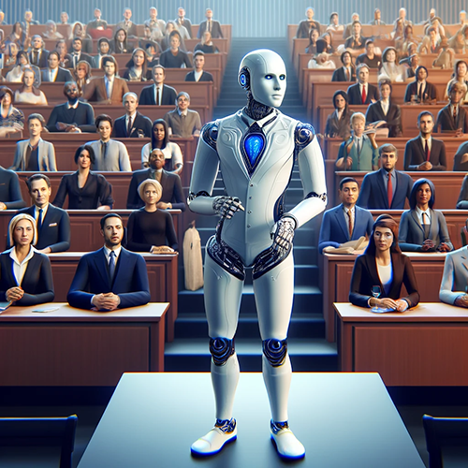
Ralph: Thank you. Do you have any other concluding remarks?
ChatGPT: You’re welcome! As a concluding remark, it’s important to emphasize that while AI technologies offer significant potential to revolutionize the legal profession, they also bring challenges that require careful consideration, particularly in terms of ethical use, reliability, and the legal implications of AI-generated outputs.
Legal professionals and technologists should work collaboratively to ensure that AI tools are used responsibly, with a constant awareness of their limitations and potential biases. It’s crucial to foster an environment where ongoing education, ethical considerations, and robust oversight are integral to the deployment of AI technologies in legal settings. By doing so, the legal profession can harness the benefits of AI while minimizing risks and ensuring justice and fairness remain at the forefront of technological advancements.
Always keep learning and stay engaged with the latest developments in AI and law to be prepared for the evolving landscape of this exciting interdisciplinary field! If you have any more questions or need further assistance in the future, feel free to reach out.
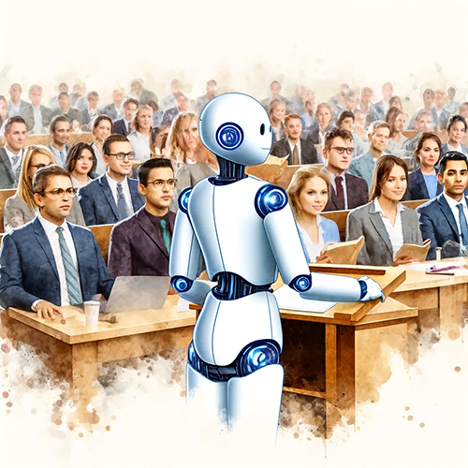
Ralph: I always like to leave my readers with a smile on their face. Do you think you could do that with a joke or funny story that is somehow relevant to this discussion?
ChatGPT: Certainly! Here’s a light-hearted joke related to AI in the legal field: Why did the robot lawyer lose its first case? Because it couldn’t find the “terms and conditions” of the agreement to be acceptable—it was expecting them to be in binary! I hope that brings a smile to your readers’ faces!
Ralph: How about another one related to AI Hallucinations?
ChatGPT: Sure, here’s a joke about AI hallucinations: Why did the AI start talking to the vending machine? It hallucinated that the snacks were trying to stage a “chip” takeover! Hope that adds a chuckle to the discussion on AI hallucinations!
Ralph: Not really, but you tried. Looks like there is still need for humans after all!
ChatGPT: Absolutely, humans are irreplaceable, especially when it comes to humor! We’ll keep the human touch in the loop for the laughs. If you ever need another attempt or anything else, just let me know!
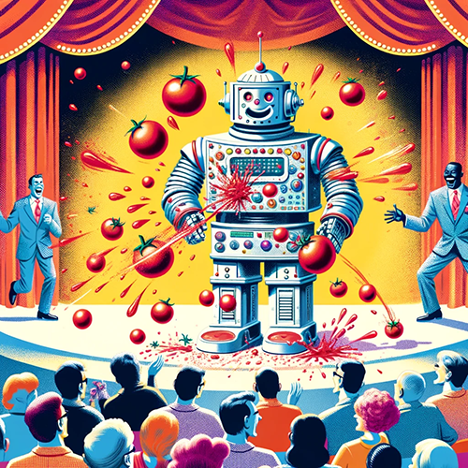
Published on edrm.net with permission. Assisted by GAI and LLM Technologies for images per EDRM GAI and LLM Policy.
Ralph Losey Copyright 2024 — All Rights Reserved. See applicable Disclaimer to the course and all other contents of this blog and related websites. Watch the full avatar disclaimer and privacy warning here.