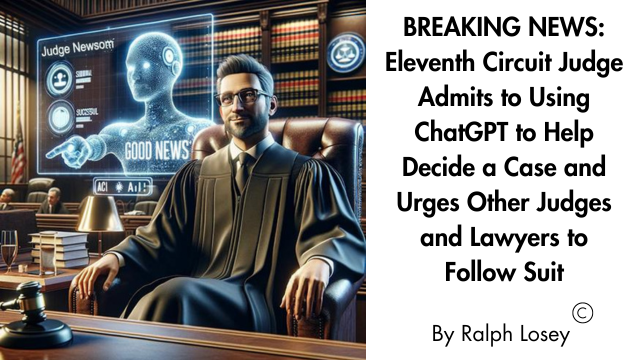
[Editor’s Note: This article was first published June 3, 2024 and EDRM is grateful to Ralph Losey for permission to republish. The opinions and positions are those of the author.]
The Eleventh Circuit published a ground breaking Concurring Opinion on May 28, 2024 by Judge Kevin C. Newsom on the use of generative AI to help decide contract interpretation issues. Snell v. United Specialty Ins. Co., 2024 U.S. App. LEXIS 12733 *; _ F.4th _ (11th Cir., 05/28/24). The case in question centered around interpretation of an insurance policy. Circuit Judge Kevin C. Newsom not only admits to using ChatGPT to help him make his decision, but praises its utility and urges other judges and lawyers to do so too. His analysis is impeccable and his writing is superb. That is bold judicial leadership – Good News. I love his opinion and bet that you will too.
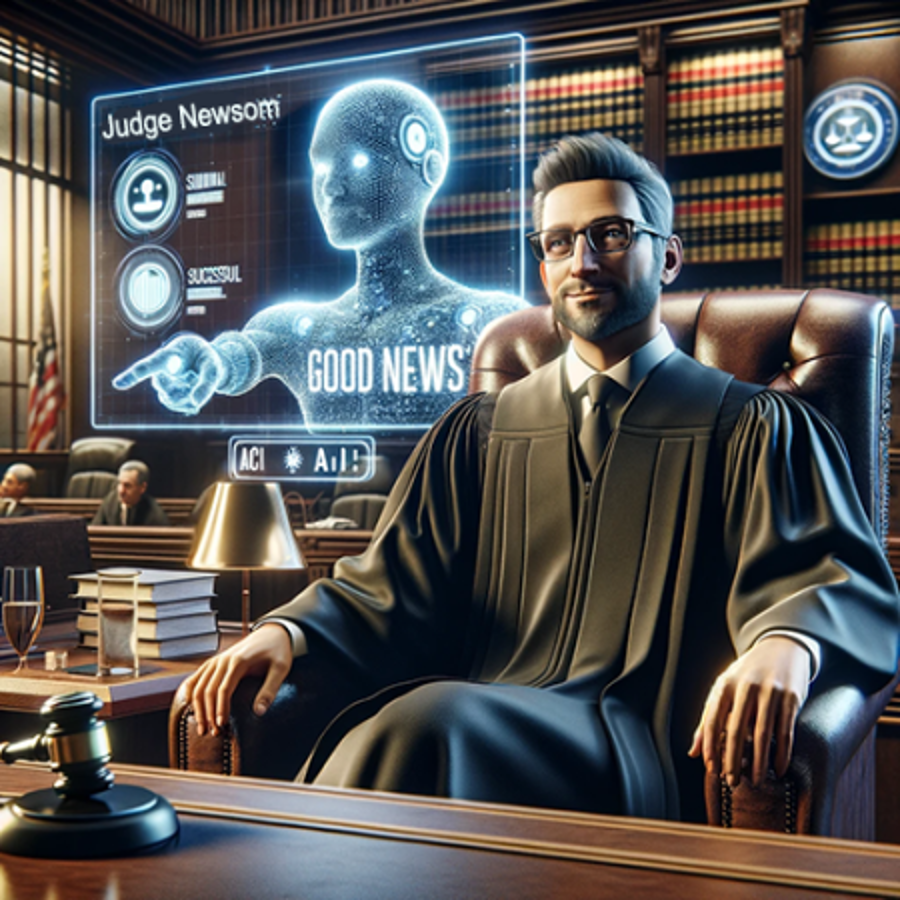
The only way to do the Concurring Opinion justice is to quote all of it, all 6,485 words. I know that’s a lot of words, but unlike ChatGPT, which is a good writer, Judge Newsom is a great writer. Judge Kevin C. Newsom, a Harvard law graduate from Birmingham, Alabama, is creative in his wise and careful use of AI. Judge Newsom added photos to his opinion and, as I have been doing recently in my articles, quoted in full the transcripts of the ChatGPT sessions he relied upon. He leads by doing and his analysis is correct, including especially his commentary on AI and human hallucinations.
Judge Newsom has an interesting, personal story to tell, and, unlike ChatGPT, he tells it in an amusing and self-effacing way. This is the first case of its kind and deserves careful study by lawyers and judges all over the world. Help me to get the word out by sharing his Concurring Opinion with your friends and colleagues. Your clients should see it too.
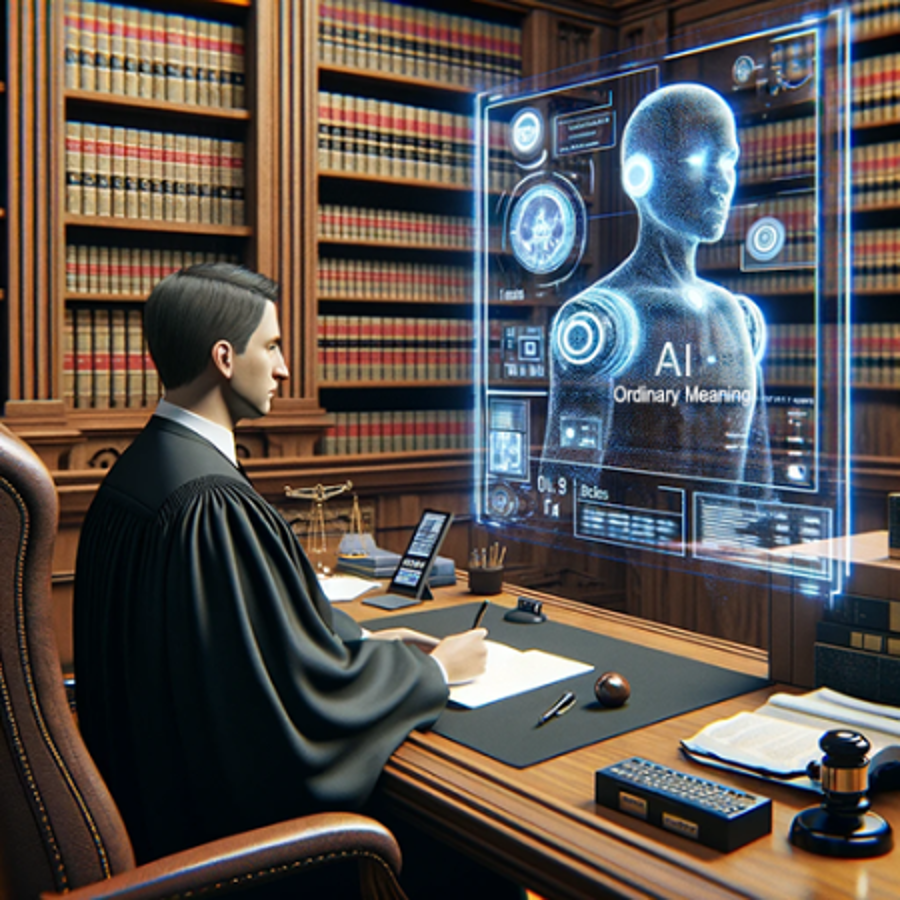
To spice it up a little, and because I can make my blogs as long as I want, which is unheard of these days, I add a few obvious editorial comments along the way (in italics), including bolding. I do this to point out a few things, and add some deserved praise of this way cool opinion.
So settle in and prepare yourself for a interesting, clever read. I promise that it will be the best concurring opinion to an insurance contract case that you have ever read. Plus, since you are probably an AI enthusiast like me, you will want to cite and quote parts of this opinion for years to come. My comments are in italics in parentheses starting with (RL-. (If you see any errors, they are mine, not Judge Newsom’s, as I rushed without assistance to get this out to you quickly.)
Newsom, Circuit Judge, concurring:
I concur in the Court’s judgment and join its opinion in full. I write separately (and I’ll confess this is a little unusual1 ) simply to pull back the curtain on the process by which I thought through one of the issues in this case—and using my own experience here as backdrop, to make a modest proposal regarding courts’ interpretations of the words and phrases used in legal instruments.
Here’s the proposal, which I suspect many will reflexively condemn as heresy, but which I promise to unpack if given the chance: Those, like me, who believe that “ordinary meaning” is the foundational rule for the evaluation of legal texts should consider—consider—whether and how AI-powered large language models like OpenAI’s ChatGPT, Google’s Gemini, and Anthropic’s Claude might—might—inform the interpretive analysis. There, having thought the unthinkable, I’ve said the unsayable.
I
First, a bit of background. [*26] As today’s majority opinion recounts, both in the district court and before us, the parties litigated this as an “ordinary meaning” case. In particular, they waged war over whether James Snell’s installation of an in-ground trampoline, an accompanying retaining wall, and a decorative wooden “cap” fit within the common understanding of the term “landscaping” as used in the insurance policy that Snell had purchased from United Specialty Insurance Company.
So, for instance, the district court observed that “whether the claims are covered depends upon whether the performance of ‘landscaping’ would include Snell’s installation of the trampoline.” Doc. 23 at 10. Because the policy didn’t define the term “landscaping,” the court said, the coverage determination turned on whether Snell’s trampoline-related work fit the “common, everyday meaning of the word.” Id. at 10-11. Having reviewed multiple dictionary definitions provided by the parties, the court concluded that Snell’s work didn’t constitute “landscaping.” Id. at 13. As the majority opinion explains, the plain-meaning battle continued on appeal, with the parties “expend[ing] significant energy parsing the words of the policy, including [*27] whether the site work necessary to install the trampoline was ‘landscaping.’” Maj. Op. at 17. Snell insisted, for example, that the district court had erred by “ignor[ing] the plain meaning of undefined terms” in the policy—most notably, “landscaping.” Br. of Appellant at 20, 21.
Now, as it turned out, we managed to resolve this case without having to delve too deeply into the definitional issue that the parties featured—due in large part to (1) a quirk of Alabama law that, according to the state supreme court, makes every insurance application ipso facto part of the policy that it precedes2 and (2) the fact that in his application Snell had expressly denied that his work included “any recreational or playground equipment construction or erection.” Maj. Op. at 17-18 (quotation marks omitted). Combined, those two premises yield the majority opinion’s controlling conclusion: “Snell’s insurance application—which Alabama law requires us to consider part of the policy—expressly disclaims the work he did here” and thus defeats his claim. Id. at 18.
Importantly, though, that off-ramp wasn’t always obviously available to us—or at least as I saw things, to me. Accordingly, I spent hours [*28] and hours (and hours) laboring over the question whether Snell’s trampoline-installation project qualified as “landscaping” as that term is ordinarily understood. And it was midway along that journey that I had the disconcerting thought that underlies this separate writing: Is it absurd to think that ChatGPT might be able to shed some light on what the term “landscaping” means? Initially, I answered my own question in the affirmative: Yes, Kevin, that is positively absurd. But the longer and more deeply I considered it, the less absurd it seemed.
But I’m getting ahead of myself. I should tell the full story, from beginning to end. In what follows, I’ll first explain how my initial efforts to pinpoint the ordinary meaning of the term “landscaping” left me feeling frustrated and stuck, and ultimately led me—initially half-jokingly, later more seriously—to wonder whether ChatGPT and other AI-powered large language models (“LLMs”) might provide a helping hand. Next, I’ll explore what I take to be some of the strengths and weaknesses of using LLMs to aid in ordinary-meaning interpretation. Finally, given the pros and cons as I see them, I’ll offer a few ideas about how we—judges, lawyers, [*29] academics, and the broader AI community—might make LLMs more valuable to the interpretive enterprise.
II
First things first. I’m unabashedly a plain-language guy—firmly of the view that “[t]he ordinary meaning rule is the most fundamental semantic rule of interpretation” and that it should govern our reading not only of “constitutions, statutes, [and] rules,” but also, as relevant here, of “private instruments.” Antonin Scalia & Bryan A. Garner, Reading Law: The Interpretation of Legal Texts 69 (2012). Accordingly, I take it as gospel truth that absent a clear indication that some idiosyncratic, specialized meaning was intended, “[w]ords are to be understood in their ordinary, everyday meanings.” Id.; accord, e.g., Shiloh Christian Ctr. v. Aspen Specialty Ins. Co., 65 F.4th 623, 629-30 (11th Cir. 2023) (Newsom, J.) (evaluating an insurance policy’s “plain language”); Heyman v. Cooper, 31 F.4th 1315, 1319-20 (11th Cir. 2022) (Newsom, J.) (evaluating a municipal ordinance’s “ordinary meaning”); Barton v. United States AG, 904 F.3d 1294, 1298-99 (11th Cir. 2018) (Newsom, J.) (evaluating a federal statute’s “ordinary meaning”).
So, following the district court’s lead, I did here what any self-respecting textualist would do when trying to assess the ordinary meaning of a particular word, here “landscaping”: I went to the dictionaries.3 In his brief, Snell had served up a buffet of definitions, ranging [*30] from Dictionary.com’s—”to improve the appearance of (an area of land, a highway, etc.) as by planting trees, shrubs, or grass, or altering the contours of the ground”—to Wikipedia’s—”any activity that modifies the visible features of an area of land.” See Br. of Appellant at 22-23. My own research revealed, in addition, that Webster’sdefined “landscaping” as “to modify or ornament (a natural landscape) by altering the plant cover,” Merriam-Webster’s Collegiate Dictionary 699 (11th ed. 2014), and that Oxforddefined it to mean “improv[ing] the aesthetic appearance of (an area) by changing its contours, adding ornamental features, or by planting trees and shrubs,” Oxford Dictionary of English 991 (3d ed. 2010).
As occasionally happens, the dictionaries left a little something to be desired. From their definitions alone, it was tough to discern a single controlling criterion. Must an improvement be natural to count as “landscaping”? Maybe, but that would presumably exclude walkways and accent lights, both of which intuitively seemed (to me, anyway) to qualify. Perhaps “landscaping” work has to be done for aesthetic reasons? That, though, would rule out, for instance, a project [*31] to regrade a yard, say away from a house’s foundation to prevent basement flooding. I once regraded my own yard, and while my efforts did nothing to improve my house’s curb appeal, they served an important functional purpose—and for what it’s worth, I definitely thought I was engaged in “landscaping.”
After languishing in definitional purgatory for a while, I decided to look at the case from a different perspective—and I do mean look. The record contains a series of photographs of Snell’s trampoline-related project. Here’s one, which shows his prep work—in particular, the empty sand pit and the below-ground retaining wall that reinforced its borders:
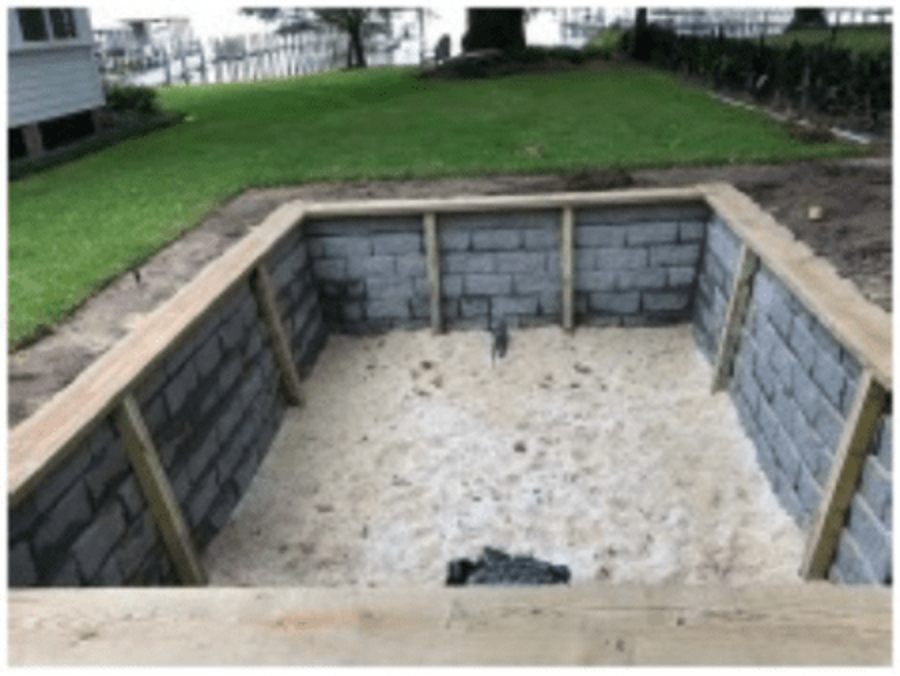
And another, which depicts the finished product, including both the polypropylene mat (the fun part) and the decorative wooden “cap”:
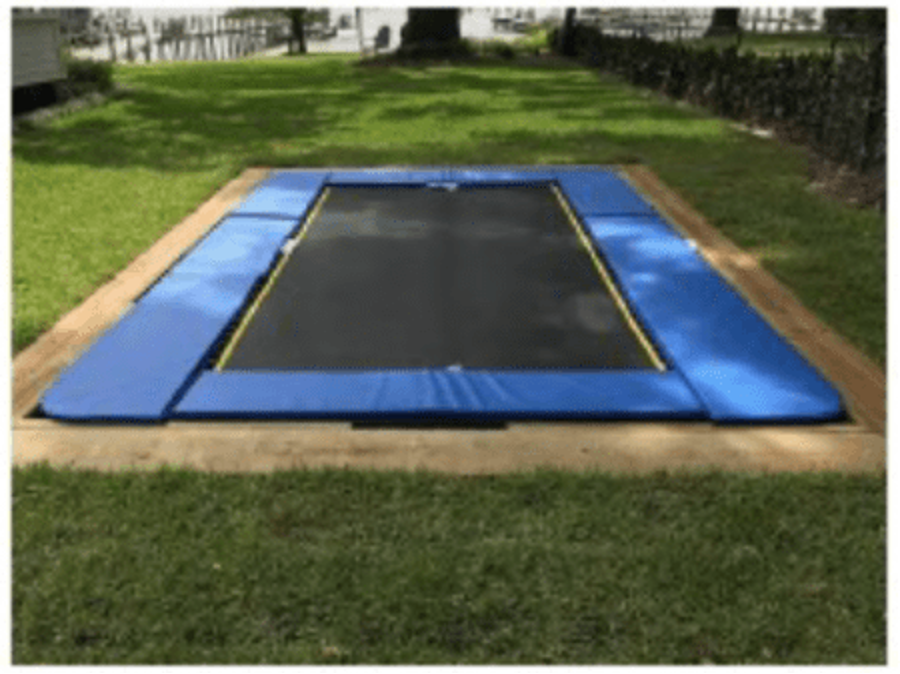
I’m not particularly proud of it, but I’ll confess that the photos affected the way I thought about the case. Nothing in them really struck me as particularly “landscaping”-y. The problem, of course, was that I couldn’t articulate why. And visceral, gut-instinct decisionmaking has always given me the willies—I definitely didn’t want to be that guy. So in a way, I felt like I was back to square one. (RL – Is Judge Newsom a great writer or what! Oddly enough, I understand exactly what he is saying here. Also note this kind of speech is way beyond ChatGPT’s ability to predict and generate, no matter what it’s temperature setting.)
And that’s [*32] when things got weird. Perhaps in a fit of frustration, and most definitely on what can only be described as a lark, I said to one of my clerks, “I wonder what ChatGPT thinks about all this.” So he ran a query: “What is the ordinary meaning of ‘landscaping’?” Here’s what ChatGPT said in response:
“Landscaping” refers to the process of altering the visible features of an area of land, typically a yard, garden or outdoor space, for aesthetic or practical purposes. This can include activities such as planting trees, shrubs, flowers, or grass, as well as installing paths, fences, water features, and other elements to enhance the appearance and functionality of the outdoor space.
Interesting, for two reasons. First, ChatGPT’s explanation seemed more sensible than I had thought it might—and definitely less nutty than I had feared. Second, it squared with my own impression—informed by my own experience writing, reading, speaking, and listening to American English in the real world—that ordinary people might well use the word “landscaping” (1) to include more than just botanical and other natural improvements and (2) to cover both aesthetic and functional objectives. In fact, several of the [*33] examples that ChatGPT flagged—”paths, fences, [and] water features”—jibed with the sorts of things that had sprung to mind when I first started thinking about the case.
Suffice it to say, my interest was piqued. But I definitely didn’t want to fall into the trap of embracing ChatGPT’s definition just because it aligned with my priors. (Bad.) So, in what might have been a mistake—more on that later—we went ahead and asked it the ultimate question: “Is installing an in-ground trampoline ‘landscaping’”? ChatGPT responded as follows:
“Yes, installing an in-ground trampoline can be considered a part of landscaping. Landscaping involves altering the visible features of an outdoor area for aesthetic or practical purposes, and adding an in-ground trampoline would modify the appearance and function of the space. It’s a deliberate change to the outdoor environment, often aimed at enhancing the overall landscape and usability of the area.”
For good measure, I posed the same questions to Google’s Bard (since replaced by Gemini).4 The precise details aren’t particularly important, but the upshot is that both models’ answers indicated that the trampoline-related work Snell had performed—the excavation [*34] of the pit, the construction of the retaining wall, the installation of the mat, and the addition of the decorative wooden cap—just might belandscaping.
As I’ve said, intervening developments—in particular, the focus on a statement in Snell’s application, which Alabama law makes part of his policy and in which Snell disclaimed doing any recreation-related work—alleviated the need to settle on the ordinary meaning of the term “landscaping.” But my own process of working through the plain-language issue was a valuable one, if only because it got me thinking about what was to me a previously unimaginable possibility: Might LLMs be useful in the interpretation of legal texts? Having initially thought the idea positively ludicrous, I think I’m now a pretty firm “maybe.” At the very least, it seems to me, it’s an issue worth exploring.
So let’s explore.
III
In what follows, I’ll try to sketch out what I take to be some of the primary benefits and risks of using LLMs—to be clear, as one implement among several in the textualist toolkit—to inform ordinary-meaning analyses of legal instruments.
A
I’ll start with the pros as I see them, and then turn to the cons.
1. LLMs train on ordinary-language [*35] inputs. (bold in original) Let me begin with what I take to be the best reason to think that LLMs might provide useful information to those engaged in the interpretive enterprise. Recall what is (for many of us, anyway) the “most fundamental semantic rule of interpretation”: Absent a clear indication that they bear some technical or specialized sense, the words and phrases used in written legal instruments “are to be understood in the ordinary, everyday meanings.” Scalia & Garner, Reading Law, supra, at 69. The premise underlying the ordinary-meaning rule is that “[i]n everyday life, the people to whom rules are addressed continually understand and apply them.” Id. at 71. Accordingly, the ordinary-meaning rule, as its name suggests, has always emphasized “common language,” Nix v. Hedden, 149 U.S. 304, 307, 13 S. Ct. 881, 37 L. Ed. 745, Treas. Dec. 14045 (1893), “common speech,” Sonn v. Magone, 159 U.S. 417, 421, 16 S. Ct. 67, 40 L. Ed. 203 (1895), and “common parlance,” Helix Energy Sols. Grp. v. Hewitt, 598 U.S. 39, 52, 143 S. Ct. 677, 214 L. Ed. 2d 409 (2023)—in short, as I’ve explained it elsewhere, “how people talk,” United States v. Caniff, 916 F.3d 929, 941 (11th Cir. 2019) (Newsom, J., concurring in part and dissenting in part), vacated and superseded, 955 F.3d 1183 (11th Cir. 2020).
The ordinary-meaning rule’s foundation in the common speech of common people matters here because LLMs are quite literally “taught” using data that aim to reflect and capture how individuals use language in their everyday lives. Specifically, the models train on a mind-bogglingly enormous [*36] amount of raw data taken from the internet—GPT-3.5 Turbo, for example, trained on between 400 and 500 billion words5 —and at least as I understand LLM design, those data run the gamut from the highest-minded to the lowest, from Hemmingway novels and Ph.D. dissertations to gossip rags and comment threads.6 Because they cast their nets so widely, LLMs can provide useful statistical predictions about how, in the main, ordinary people ordinarily use words and phrases in ordinary life.7 So, for instance, and as relevant here, LLMs can be expected to offer meaningful insight into the ordinary meaning of the term “landscaping” because the internet data on which they train contain so many uses of that term, from so many different sources—e.g., professional webpages, DIY sites, news stories, advertisements, government records, blog posts, and general online chatter about the topic.8
To be sure, LLMs’ training data aren’t a perfect [*37] universe from which to draw hard-and-fast conclusions about ordinary meaning, principally because they don’t capture what I’ll call “pure offline” usages—i.e., those that neither (1) occur online in the first instance nor (2) originate offline, in hard copy, but are eventually digitized and uploaded to some online site. And indeed, the absence of offline usages from the training pool—and in particular, the implications for underrepresented populations—strikes me as a sufficiently serious concern that I’ve broken it out for separate discussion below. See infra at 21-23. Even so, those omissions aside, it seems to me scarcely debatable that the LLMs’ training data are at the very least relevant to the ordinary-meaning analysis. In fact, an LLMs’ dataset may well be the most “perfectly imperfect” on offer because (1) scads of people either use the internet or create content that finds its way onto the internet (or more likely both), (2) the information available online reflects people’s use of terminology in a wide array of contexts and settings, from the sublime to the ridiculous, and (3) there’s little reason (that I can think of) to worry that writers and speakers whose communications [*38] end up online manipulate the inputs (i.e., their words) in a way that might artificially skew the data.
Put simply, ordinary-meaning interpretation aims to capture how normal people use language in their everyday lives—and the bulk of the LLMs’ training data seem to reflect exactly that.9
2. LLMs can “understand” context. So far as I can tell, researchers powering the AI revolution have created, and are continuing to develop, increasingly sophisticated ways to convert language (and I’m not making this up) into math that computers can “understand.” See Yonathan A. Arbel & David A. Hoffman, Generative Interpretation, 99 N.Y.U. L. Rev. (forthcoming 2024) (manuscript at 26) (describing “attention mechanism,” a feature of LLMs that facilitates the recognition of how words are used in context). The combination of the massive datasets used for training and this cutting-edge “mathematization” of language enables LLMs to absorb and assess the use of terminology in context and empowers them to detect language patterns at a granular level. So, for instance, modern LLMs can easily discern the difference—and distinguish—between the flying-mammal “bat” that uses echolocation and may or may not be living in your attic, on the one hand, [*39] and the wooden “bat” that Shohei Otani uses to hit dingers, on the other. See id.And that, as I understand it, is just the tip of the iceberg. LLM predictions about how we use words and phrases have gotten so sophisticated that they can (for better or worse) produce full-blown conversations, write essays and computer code, draft emails to co-workers, etc. And as anyone who has used them can attest, modern LLMs’ results are often sensible—so sensible, in fact, that they can border on the creepy. Now let’s be clear, LLMs aren’t perfect—and again, we’ll discuss their shortcomings in due course. But let’s be equally clear about what they are: high-octane language-prediction machines capable of probabilistically mapping, among other things, how ordinary people use words and phrases in context. (RL – Excellent reasoning here by Judge Newsom, again I think he’s got it right. Kudos to him and his clerks.)
3. LLMs are accessible. LLMs are readily accessible (and increasingly so) to judges, lawyers, and, perhaps most importantly, ordinary citizens. In recent years, the use of LLMs has proliferated, and as with all other internet-related tools, one can only assume that usage will continue to accelerate, likely at an exponential rate. The LLMs’ easy accessibility is important in at least two respects. [*40] First, it offers the promise of “democratizing” the interpretive enterprise, both (as already explained) by leveraging inputs from ordinary people and by being available for use by ordinary people. Second, it provides judges, lawyers, and litigants an inexpensive research tool. My “landscaping”-related queries, for instance, while no doubt imperfect, cost me nothing. To be sure, querying a more advanced LLM may come with a pricetag, at least for now. But so does, for example, searching the Oxford English Dictionary, the online version of which exists behind a paywall.10 And I’d be willing to bet that the costs associated with even the more advanced LLMs pale in comparison to subscriptions for Westlaw and Lexis, which power most modern legal research, including some involving dictionaries.11 And of course there’s always the promise that open-source LLMs might soon approximate the for-profit models’ productivity.
4. LLM research is relatively transparent. Using LLMs to facilitate ordinary-meaning interpretation may actually enhance the transparency and reliability of the interpretive enterprise itself, at least vis-à-vis current [*41] practice. Two brief observations.
First, although we tend to take dictionaries for granted, as if delivered by a prophet, the precise details of their construction aren’t always self-evident. Who exactly compiles them, and by what criteria do the compilers choose and order the definitions within any given entry? To be sure, we’re not totally in the dark; the online version of Merriam-Webster‘s, for instance, provides a useful primer explaining “[h]ow . . . a word get[s] into” that dictionary.12 It describes a process by which human editors spend a couple of hours a day “reading a cross section of published material” and looking for new words, usages, and spellings, which they then mark for inclusion (along with surrounding context) in a “searchable text database” that totals “more than 70 million words drawn from a great variety of sources”—followed, as I understand things, by a step in which a “definer” consults the available evidence and exercises his or her judgment to “decide[] . . . the best course of action by reading through the citations and using the evidence in them to adjust entries or create new ones.”13
Such explainers [*42] aside, Justice Scalia and Bryan Garner famously warned against “an uncritical approach to dictionaries.” Antonin Scalia & Bryan A. Garner, A Note on the Use of Dictionaries, 16 Green Bag 2d 419, 420 (2013). They highlighted as risks, for instance, that a volume could “have been hastily put together by two editors on short notice, and very much on the cheap,” and that without “consult[ing] the prefatory material” one might not be able “to understand the principles on which the dictionary [was] assembled” or the “ordering of [the] senses” of a particular term. Id. at 420, 423.
To be clear, I’m neither a nihilist nor a conspiracy theorist, but I do think that we textualists need to acknowledge (and guard against the fact) that dictionary definitions present a few known unknowns.See id. at 419-28; cf. Thomas R. Lee & Stephen C. Mouritsen, The Corpus and the Critics, 88 U. Chi. L. Rev. 275, 286-88 (2021) (highlighting potential interpretive pitfalls associated with dictionaries). And while I certainly appreciate that we also lack perfect knowledge about the training data used by cutting-edge LLMs, many of which are proprietary in nature, see supra notes 6 & 8, I think it’s fair to say that we do know both (1) what LLMs are learning from—namely, tons and tons of internet data—and (2) one of the things that makes LLMs so useful—namely, their ability [*43] to accurately predict how normal people use language in their everyday lives.
A second transparency-related thought: When a judge confronts a case that requires a careful assessment of a word’s meaning, he’ll typically consult a range of dictionary definitions, engage in a “comparative weighing,” Scalia & Garner, A Note, supra, at 422, and, in his written opinion, deploy one, two, or a few of them. The cynic, of course, will insist that the judge just dictionary-shopped for the definitions that would enable him to reverse-engineer his preferred outcome. See James J. Brudney & Lawrence Baum, Oasis or Mirage: The Supreme Court’s Thirst for Dictionaries in the Rehnquist and Roberts Eras, 55 Wm. & Mary L. Rev. 483, 539 (2013). I’m not so jaded; I trust that ordinary-meaning-focused judges genuinely seek out definitions that best fit the context of the instruments that they’re charged with interpreting. See, e.g., Hoever v. Marks, 993 F.3d 1353, 1366-68 (11th Cir. 2021) (en banc) (Newsom, J., concurring in judgment in part and dissenting in part) (choosing, based on contextual clues, from among competing definitions of the word “for”). Even so, I have to admit (1) that the choice among dictionary definitions involves a measure of discretion and (2) that judges seldom “show their work”—that is, they rarely explain in [*44] any detail the process by which they selected one definition over others. Contrast my M.O. in this case, which I would recommend as a best practice: full disclosure of both the queries put to the LLMs (imperfect as mine might have been) and the models’ answers.
Anyway, I don’t mean to paint either too grim a picture of our current, dictionary-centric practice—my own opinions are chock full of dictionary definitions, I hope to good effect—or too rosy a picture of the LLMs’ potentiality. My point is simply that I don’t think using LLMs entails any more opacity or involves any more discretion than is already inherent in interpretive practices that we currently take for granted—and in fact, that on both scores it might actually involve less.
5. LLMs hold advantages over other empirical interpretive methods. One final point before moving on. Recently, some empiricists have begun to critique the traditional dictionary-focused approach to plain-meaning interpretation. Some, for instance, have conducted wide-ranging surveys of ordinary citizens, seeking to demonstrate that dictionaries don’t always capture ordinary understandings of legal texts. See, e.g., Kevin P. Tobia, Testing Ordinary Meaning [*45] , 134 Harv. L. Rev. 726 (2020). Others have turned to corpus linguistics, which aims to gauge ordinary meaning by quantifying the patterns of words’ usages and occurrences in large bodies of language. See, e.g., Thomas R. Lee & Stephen C. Mouritsen, Judging Ordinary Meaning, 127 Yale L.J. 788, 795 (2018).
On balance, reliance on LLMs seems to me preferable to both. The survey method is interesting, but it seems wildly impractical—judges and lawyers have neither the time nor the resources to poll ordinary citizens on a widespread basis. By contrast, as already explained, LLMs are widely available and easily accessible. And corpus methods have been challenged on the ground, among others, that those tasked with compiling the data exercise too much discretion in selecting among the inputs. See, e.g., Jonathan H. Choi, Measuring Clarity in Legal Text, 91 U. Chi. L. Rev. 1, 26 (2024). For reasons already explained, I don’t think LLM-based methods necessarily carry the same risk.
For all these reasons, and perhaps others I haven’t identified, it seems to me that it’s at least worth considering whether and how we might leverage LLMs in the ordinary-meaning enterprise—again, not as the be all and end all, but rather as one aid to be used alongside dictionaries, the semantic canons, [*46] etc.
B
Now, let’s examine a few potential drawbacks. I suppose it could turn out that one or more of them are deal-killers. I tend to doubt it, but let’s put them on the table.
1. LLMs can “hallucinate.” First, the elephant in the room: What about LLMs’ now-infamous “hallucinations”? Put simply, an LLM “hallucinates” when, in response to a user’s query, it generates facts that, well, just aren’t true—or at least not quite true. See, e.g., Arbel & Hoffman, supra, at 48-50. Remember the lawyer who got caught using ChatGPT to draft a brief when it ad-libbed case citations—which is to say cited precedents that didn’t exist? See, e.g., Benjamin Weiser, Here’s What Happens When Your Lawyer Uses ChatGPT, N.Y. Times (May 29, 2023). To me, this is among the most serious objections to using LLMs in the search for ordinary meaning. Even so, I don’t think it’s a conversation-stopper. For one thing, LLM technology is improving at breakneck speed, and there’s every reason to believe that hallucinations will become fewer and farther between. Moreover, hallucinations would seem to be most worrisome when asking a specific question that has a specific answer—less so, it seems to me, when more generally seeking the “ordinary meaning” [*47] of some word or phrase. Finally, let’s shoot straight: Flesh-and-blood lawyers hallucinate too. Sometimes, their hallucinations are good-faith mistakes. But all too often, I’m afraid, they’re quite intentional—in their zeal, attorneys sometimes shade facts, finesse (and even omit altogether) adverse authorities, etc. So at worst, the “hallucination” problem counsels against blind-faith reliance on LLM outputs—in exactly the same way that no conscientious judge would blind-faith rely on a lawyer’s representations. (RL – I love this part about human lawyers also hallucinating. This corresponds with my own experience as I have written before. I plan to quote this often. Pretty soon ChatGPT will be able to predict it!)
2. LLMs don’t capture offline speech, and thus might not fully account for underrepresented populations’ usages.I flagged this one earlier, but I think it’s a serious enough concern to merit separate treatment. Here’s the objection, as I see it: People living in poorer communities (perhaps disproportionately minorities and those in rural areas) are less likely to have ready internet access and thus may be less likely to contribute to the sources from which LLMs draw in crafting their responses to queries. Accordingly, the argument goes, their understandings—as manifested, for instance, in their written speech—won’t get “counted” in the LLMs’ ordinary-meaning assessment.
As [*48] I say, I think this is a serious issue. Even so, I don’t believe it fatally undermines LLMs’ utility, at least as one tool among many for evaluating ordinary meaning. Ideally, of course, the universe of information from which any source of meaning draws would capture every conceivable input. But we should guard against overreaction. Presumably, LLMs train not only on data that were born (so to speak) online but also on material that was created in the physical world and only thereafter digitized and uploaded to the internet. And there is (I think) less reason to fear that those in underserved communities are at a dramatic comparative disadvantage with respect to the latter category.Moreover, to the extent we’re worried about a lack of real-world, documentary evidence representing underrepresented populations’ usages, then we have bigger fish to fry, because there’s reason to doubt the utility of dictionaries, as well—which, as Merriam-Webster‘s editors have explained, also rely on hard-copy sources to evaluate terms’ ordinary meanings. See supraat 16-17 & note 12. (RL – I agree with Judge Newsom’s commendable concerns here about bias of sorts built into the daya, but like him, agree that in this legal situation at least, there is no reason for concern.)
Anyway, the risk that certain communities’ word-usage outputs aren’t adequately reflected in LLMs’ training-data inputs [*49] is real, and I’d note it as a candidate for improvement, but I don’t think it’s either fatal or insurmountable.14
3. Lawyers, judges, and would-be litigants might try to manipulate LLMs. I suppose there’s a risk that lawyers and judges might try to use LLMs strategically to reverse-engineer a preferred answer—say, by shopping around among the available models or manipulating queries. Maybe, but that’s an evergreen issue, isn’t it? Although they shouldn’t, lawyers and judges can cast about for advantageous dictionary definitions and exploit the interpretive canons, but no one thinks that’s a sufficient reason to abandon those as interpretive tools. And if anything, I tend to think that the LLMs are probably less vulnerable to manipulation than dictionaries and canons, at least when coupled with (as I’ve tried to provide here) full disclosure of one’s research process. (RL- Very clever observation. It is also important to acknowledge that Judge Newsom is being fully transparent in his disclosure of use of AI. In fact, this is one of the most transparent and personally revealing opinions I have ever read.)
Relatedly, might prospective litigants seek to corrupt the inputs—the data on which the LLMs train and base their responses to user queries—in an effort to rig the system to spit out their preferred interpretations? It’s a real concern—perhaps especially considering that the same AI companies that have developed and [*50] are training the LLMs might themselves be litigants. But given the nature of the technology as I understand it, hardly insurmountable. For one thing, most models embody some training “cutoff”—for instance, though things might have changed, it was once common knowledge that GPT-4 learned on data up to and including September 2021. See Open AI, GPT-4 Technical Report 10 (arXiv:2303.08774, 2024). Accordingly, it would likely be difficult, if not impossible, to pollute the inputs retroactively. More fundamentally, it seems almost inconceivable that a would-be malefactor could surreptitiously flood any given dataset with enough new inputs to move the needle—remember, just by way of example, that GPT-3.5 Turbo trained on more than 400 billion words. Finally, while I tend to doubt that any AI company would conclude that corrupting its own product in order to obtain an interpretive advantage in a single case was in its long-term business interest, that risk, it seems to me, could be mitigated, if not eliminated, by querying multiple models rather than just one. (RL – agree with this observation and the Judge’s conclusions.)
4. Reliance on LLMs will lead us into dystopia. Would the consideration of LLM outputs in interpreting legal texts inevitably put us [*51] on some dystopian path toward “robo judges” algorithmically resolving human disputes? I don’t think so. As Chief Justice Roberts recently observed, the law will always require “gray area[]” decisionmaking that entails the “application of human judgment.” Chief Justice John G. Roberts, Jr., 2023 Year-End Report on the Federal Judiciary 6 (Dec. 31, 2023). And I hope it’s clear by this point that I am not—not, not, not—suggesting that any judge should ever query an LLM concerning the ordinary meaning of some word (say, “landscaping”) and then mechanistically apply it to her facts and render judgment. My only proposal—and, again, I think it’s a pretty modest one—is that we consider whether LLMs might provide additional datapoints to be used alongside dictionaries, canons, and syntactical context in the assessment of terms’ ordinary meaning. That’s all; that’s it. (RL – My only criticism of Judge Newsom’s Concurring Opinion is that he does not go further. I for one think judges should go much further in their use of generative AI, as I have written about previously. Appellate judges may be among the first to be routinely supplemented. See e.g. Circuits in Session: How AI Challenges Traditional Appellate Dynamics (e-Discovery Team, 10/13/23); Circuits in Session: Addendum and Elaboration of the Appellate Court Judge Experiment (e-Discovery Team, 10/26/23); Circuits in Session: Analysis of the Quality of ChatGPT4 as an Appellate Court Judge (e-Discovery Team, 11/01/23). But I understand why Judge Newsom does not do that here. One step at a time and this opinion is an important first step.)
IV
Which brings me to my final question: If I’m not all wet, and it’s at least worth considering whether LLMs have a role to play in the interpretation of legal instruments, how might we maximize their utility? I’ve already flagged a few suggestions for improvement along the way—more data, from more sources, representing a more [*52] representative cross-section of Americans. But beyond the obvious, what else? (RL – No Judge Newsom, you are not all wet. You are squeaky clean, on point and over-modest. If only we had more judges like you.Certainly LLMs have a very important role to play in the interpretation of legal instruments.)
First, I think it’ll be helpful to clarify the objective. Remember that in my clumsy first crack at this, I asked two different models two different questions: (1) “What is the ordinary meaning of ‘landscaping’?”; and (2) “Is an in-ground trampoline ‘landscaping’?” Which is the proper question? In retrospect, if my contention is—as it is—that LLMs might aid in the search for the ordinary, everyday meaning of common words and phrases, then it seems pretty clear to me that my first, more general query is the more appropriate one. The models’ highest and best use is (like a dictionary) helping to discern how normal people use and understand language, not in applying a particular meaning to a particular set of facts to suggest an answer to a particular question.
Second, and relatedly, how can we best query LLMs? Those in the know refer to the question a user asks a model as a “prompt.” I’ll confess that I gave relatively little thought to my own prompts—they were just the questions that immediately sprang to mind. But research indicates that the models can be sensitive to prompts and that the results can vary accordingly. [*53] See, e.g., Arbel & Hoffman, supra, at 36. So it may be wise for users to try different prompts, and, importantly, to report the prompts they use and the range of results they obtain. Id. at 36-37. Better still to do all that andquery multiple models to ensure that the results are consistent—or, in statistics-speak, “robust.” (RL – How can we best query LLM’s? That is indeed the key question of the day and the whole idea behind Prompt Engineering, a subject that has been the focus of my studies and experiments for some time now. I promise you Judge Newsom that many have been working hard on this challenge and should have a solution for this soon.)
Third, we need to clarify the particular output we’re after. The questions I asked sought a discrete, one-time answer. In particular, I asked for a single definition of “landscaping” and, separately, whether installation of an in-ground trampoline qualified. One potential challenge is that this approach obscures the fact, already explained, that LLMs make probabilistic, predictive judgments about language. With that in mind, some who have considered how LLMs might be used to interpret contracts have suggested that users seek not just answers but also “confidence” levels. See id. at 23. So, for instance, an LLM might reveal that its prediction about a provision’s meaning is “high” or, by contrast, only “ambiguous.” Alternatively, but to the same end, a researcher might ask an LLM the same question multiple times and note the percentage of instances in which it agrees that, say, installation of an in-ground [*54] trampoline is landscaping. See Christoph Engel & Richard H. McAdams, Asking GPT for the Ordinary Meaning of Statutory Terms 15 (Max Planck Inst. Discussion Paper 2024/5).15 (RL – Yes, asking multiple times is one way of many to improve the quality of the AI input. Again that is a question of prompt engineering.)
Fourth and finally, there are temporal considerations to mull. The ordinary-meaning rule has an important corollary—namely, that “[w]ords must be given the meaning they had when the text was adopted.” Scalia & Garner, Reading Law, supra, at 78 (emphasis added). That principle—”originalism,” if you will—most obviously applies to constitutional and statutory texts. See, e.g., United States v. Pate, 84 F.4th 1196, 1201 (11th Cir. 2023) (en banc) (“[W]hen called on to resolve a dispute over a statute’s meaning, [a court] normally seeks to afford the law’s terms their ordinary meaning at the time Congress adopted them.” (quoting Niz-Chavez v. Garland, 593 U.S. 155, 160, 141 S. Ct. 1474, 209 L. Ed. 2d 433 (2021)). To be clear, though, it holds for private instruments like contracts and insurance policies, as well. See Scalia & Garner, Reading Law, supra, at 78. This case didn’t present a particularly knotty temporal difficulty because the policy in question was issued relatively recently, in 2017. But cases routinely arise in which the relevant question is what a particular term meant in the more distant past—say, in 1787, 1868, or 1964. If LLMs are to be deployed to aid more broadly in the search for ordinary meaning, it would be enormously [*55] helpful—if it’s not already possible—for AI engineers to devise a way in which queries could be limited to particular timeframes. Cf. Engel & McAdams, supra, at 34-37.16 (RL – Agree this would be very possible and I understand the constitution construction hints and implications here.)
V
In his most recent year-end report on the state of the federal judiciary, Chief Justice Roberts cautioned that the “use of AI requires caution and humility.” Roberts, supra, at 5. I wholeheartedly agree. Importantly, though, I also agree with what I take to be the report’s assumption that AI is here to stay. Now, it seems to me, is the time to figure out how to use it profitably and responsibly. It’s in that spirit that I’ve offered these preliminary thoughts about whether and how LLMs might aid lawyers and judges in the interpretive enterprise. Plenty of questions remain, and I’m sure I haven’t even identified all of them. But—and this is my bottom line—I think that LLMs have promise. At the very least, it no longer strikes me as ridiculous to think that an LLM like ChatGPT might have something useful to say about the common, everyday meaning of the words and phrases used in legal texts.
Appendix
Below are the questions that I asked OpenAI’s ChatGPT and Google’s Bard models, along with their responses.
ChatGPT
Question: What is the ordinary meaning of “landscaping” [*56] ?
Response: “Landscaping” refers to the process of altering the visible features of an area of land, typically a yard, garden or outdoor space, for aesthetic or practical purposes. This can include activities such as planting trees, shrubs, flowers, or grass, as well as installing paths, fences, water features, and other elements to enhance the appearance and functionality of the outdoor space.
Question: Is installing an in-ground trampoline “landscaping”?
Response: Yes, installing an in-ground trampoline can be considered a part of landscaping. Landscaping involves altering the visible features of an outdoor area for aesthetic or practical purposes, and adding an in-ground trampoline would modify the appearance and function of the space. It’s a deliberate change to the outdoor environment, often aimed at enhancing the overall landscape and usability of the area.
Bard
Question: What is the ordinary meaning of “landscaping”?
Response: The ordinary meaning of landscaping” is the process of modifying the visible features of an area of land, including:
• Adding or removing vegetation, such as trees, shrubs, and flowers
• Installing hardscape elements, such as walkways, patios, and retaining walls [*57]
• Creating or enhancing natural features, such as ponds, waterfalls, and rock gardens
Landscaping can be used to improve the aesthetics of an area, increase its functionality, and/or protect it from erosion. It can also be used to create a sense of privacy, define spaces, and add value to a property.
Here are some examples of landscaping projects:
• Planting a new lawn or garden
• Installing a sprinkler system
• Creating a pond or waterfall
Landscaping can be a DIY project, or it can be hired out to a professional landscaping company. The cost of landscaping will vary depending on the size and scope of the project.
Question: Is installing an in-ground trampoline “landscaping”?
Response: Whether or not installing an in-ground trampoline is considered landscaping depends on how you define the term. If you define landscaping broadly to include any modification to the visible features of an area of land, then installing an in-ground trampoline would be considered landscaping. However, if you define landscaping more narrowly to focus on the planting and maintenance [*58] of vegetation, then installing an in-ground trampoline would not be considered landscaping.
Here is a table that summarizes the different ways to define landscaping and whether or not installing an in-ground trampoline would be considered landscaping under each definition:
Definition | Includes installing an in- |
ground trampoline? | |
Broad definition: Any | Yes |
modification to the visible | |
features of an area of land | |
Narrower definition: Planting | No |
and maintenance of vegetation |
Ultimately, whether or not installing an in-ground trampoline is considered landscaping is a matter of opinion. There is no right or wrong answer.
End of Document
1 Even for me.
2 For what it’s worth, I don’t think the governing Alabama statute says that. In relevant part, it provides that “[e]very insurance contract shall be construed according to the entirety of its terms and conditions as set forth in the policy and as . . . modified by any rider, endorsement or application which is part of the policy.” Ala. Code § 27-14-17(a). The absence of a comma before the clause “which is part of the policy” strongly indicates, to my mind, that an insured’s “application” should be deemed to “modif[y]” the contract only if it is made “part of the policy.” Be that as it may, as the majority opinion correctly notes, the Alabama Supreme Court has read § 27-14-17(a), in effect, to incorporate every insured’s application into the terms of his policy, and we’re stuck with that interpretation, even if erroneous. See Maj. Op. at 18 (citing Atlanta Cas. Co. v. Russell, 798 So. 2d 664, 667 (Ala. 2001)).
3 Alabama law governs the interpretation of the insurance contract at issue in this case, see St. Paul Fire & Marine Ins. Co. v. ERA Oxford Realty Co. Greystone, LLC, 572 F.3d 893, 894 n.1 (11th Cir. 2009), and privileges “ordinary meaning” in that endeavor, see Safeway Ins. Co of Alabama v. Herrera, 912 So. 2d 1140, 1144 (Ala. 2005).
4 Generally, Bard’s response to my general question—”What is the ordinary meaning of ‘landscaping’?”—was pretty similar to ChatGPT’s, though notably longer. When asked the more specific question—”Is installing an in-ground trampoline ‘landscaping’?”—Bard was more equivocal than ChatGPT had been. I’ve included my questions and the models’ responses in an appendix for readers’ reference.
5 See Christoph Engel & Richard H. McAdams, Asking GPT for the Ordinary Meaning of Statutory Terms 10-11 (Max Planck Inst. Discussion Paper 2024/5).
6 I’ll confess to a bit of uncertainty about exactly what data LLMs use for training. This seems like an area ripe for a transparency boost, especially as LLMs become increasingly relevant to legal work. But here’s what I think I’ve gathered from some sleuthing. A significant chunk of the raw material used to train many LLMs—i.e., the “stuff” from which the models learn—comes from something called the Common Crawl, which is, in essence, a massive data dump from the internet. See, e.g., Yiheng Liu, et al., Understanding LLMs: A Comprehensive Overview from Training to Inference 6-8 (arXiv:2401.02038, 2024). The Common Crawl isn’t “the entire web”; rather, it’s a collection of samples from online sites, which AI companies further refine for training purposes. SeeStefan Baack, Training Data for the Price of a Sandwich: Common Craw’s Impact on Generative AI 5, 16-24, Mozilla Insights (Feb. 2024). That said, the samples are massive. (RL – Yes, that is a large part, but not all of it, and most agree with Judge Newsom that greater transparency is required from OpenAI and other vendors on this issue.)
7 To be clear, I do mean “predictions.” As I understand things, the LLM that underlies a user interface like ChatGPT creates, in effect, a complex statistical “map” of how people use language—that, as machine-learning folks would say, is the model’s “objective function.” How does it do it? Well, to dumb it way down, drawing on its seemingly bottomless reservoir of linguistic data, the model learns what words are most likely to appear where, and which ones are most likely to precede or follow others—and by doing so, it can make probabilistic, predictive judgments about ordinary meaning and usage. SeeYonathan A. Arbel & David A. Hoffman, Generative Interpretation, 99 N.Y.U. L. Rev. (forthcoming 2024) (manuscript at 24-29); Engel & McAdams, supra, at 10-11. (RL – Sounds correct to me.)
8 So far as I understand things, it’s next to impossible to pinpoint exactly what training data an LLM draws on when answering a particular question, but from what I’ve seen, I think it’s fair to say that it’s a pretty wide cross-section.
9 I’ll bracket for the time being whether LLMs might be useful (or less so) in the fraction of cases in which we’re focused on technical or specialized meaning, rather than ordinary meaning. See Scalia & Garner, Reading Law, supra, at 73.
10 See Purchase, Oxford English Dictionary, https://www.oed.com/purchase (last visited May 23, 2024).
11 Westlaw, for instance, allows paid subscribers to access the latest edition of Black’s Law Dictionary. Lexis permits its users to access similar offerings, including Ballentine’s Law Dictionary.
12 Help: How does a word get into a Merriam-Webster dictionary?, Merriam-Webster (last visited May 23, 2024), https://www.merriam-webster.com/help/faq-words-into-dictionary [https://perma.cc/446C-WYMN].
13 Id.
14 A quasi-related issue: Some words have acquired “regionalized” meanings over time. So, for instance, the noun “toboggan” can refer to either (1) a “long flat-bottomed light sled,” (2) a “downward course or sharp decline,” or (3) a “stocking cap.” Merriam-Webster’s Collegiate Dictionary, supra, at 1313. Notably, though, the third sense is “chiefly Southern [and] Midland.” Id. When we asked ChatGPT, “What is the ordinary meaning of ‘toboggan’?”, it responded with only the first, sled-based explanation. The lesson is simply that interpreters using LLMs for assistance would be wise to remember, as always, that “context is king,” Wachovia Bank, N.A. v. United States, 455 F.3d 1261, 1267 (11th Cir. 2006), and, accordingly, that they might need to adjust their queries to account for its influence.
15 Some might worry that seeking a range of responses could cause the LLM to respond with uncommon usages. Of course, if the rogue results are rare, then, almost by definition, they won’t move the “ordinary meaning” needle. And if, by contrast, they’re not rare—and thus aren’t rogues at all—then perhaps they indicate that we need to rethink our intuitions about what the “ordinary meaning” really is. Fine, and good.
16 Relatedly, might we have a “start date” problem? Are we limited to ordinary understandings that post-date the launch of the internet? Or might it be that the information contained on the internet is so extensive that it can aid in understanding historical usages, as well?
Republished on edrm.net with permission. Assisted by GAI and LLM Technologies for images per EDRM GAI and LLM Policy.
Ralph Losey Copyright 2024 (excluding the court opinion) — All Rights Reserved. See applicable Disclaimer to the course and all other contents of this blog and related websites. Watch the full avatar disclaimer and privacy warning here.