An Introduction to Large Language Models for Smart Ediscovery Professionals
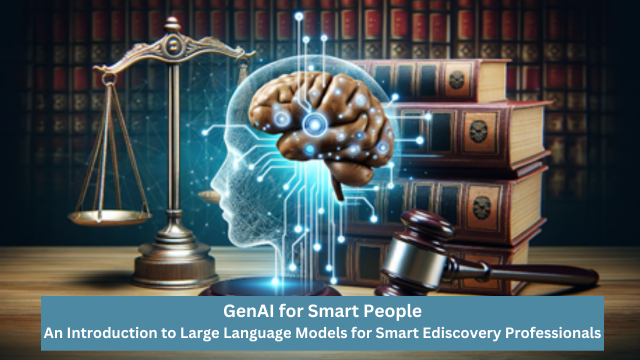
[EDRM Editor’s Note: The opinions and positions are those of John Tredennick and Dr. William Webber.]
It’s been more than a year since ChatGPT upended our thinking about artificial intelligence. Over that time, discussions about Large Language Models like GPT (the engine behind ChatGPT) have taken center stage in just about every field of endeavor, but especially in the legal profession. At the recent LegalWeek conference, nearly every vendor on the exhibit floor touted their new Generative AI integrated software–or at least talked about how GenAI would be added to their product “real soon.”
This Guide provides an introduction to Generative AI for smart legal professionals. While someone will no doubt release a “GenAI book for Dummies” in the near future, we don’t see dummies in our profession. Rather, it is filled at all levels with smart people, many of whom would like to better understand how GenAI can make their practices more efficient and effective. We hope you enjoy it.
We can also use LLMs to review and analyze groups of documents… There are different ways to accomplish this task but our approach is representative of a process called “RAG” or Retrieval Augmented Generation.
John Tredennick and Dr. William Webber, Merlin Search Technologies.
Executive Summary “GenAI for Smart People,” authored by John Tredennick and Dr. William Webber, serves as an essential guide for legal professionals navigating the integration of Generative AI (GenAI) into eDiscovery workflows. The article breaks down complex concepts into digestible insights, offering a forward-looking perspective on the technology’s transformative impact. Introduction to GenAI: The guide introduces GenAI, emphasizing its pivotal role in enhancing legal practices. It sheds light on how GenAI, particularly through Large Language Models (LLMs) like GPT, has become central to discussions in the legal field, promising to streamline processes and improve efficiency. Role of LLMs: The authors elaborate on LLMs’ functionality, focusing on their ability to process and generate text based on extensive training. This section underscores the computational prowess and the intricate technology driving these models, highlighting their significance in text-based applications. Training and Operational Mechanics: The paper details the rigorous training LLMs undergo, including reinforcement learning and the implications of a fixed knowledge base post-training cutoff. It brings attention to the models’ limitations and the ongoing debate around their ability to truly “understand” content versus merely replicating it. Application in eDiscovery:The authors demonstrate LLMs’ capability to revolutionize document review, analysis, and transcript review by rapidly identifying relevant information, significantly cutting down the time and resources required for these tasks.Through practical examples, the article showcases how GenAI can offer immediate positive effects on discovery practices, despite the architectural limits of current models. Data Security: Addressing concerns around data privacy and the protection of attorney-client privileges, the guide reassures readers about the robust security measures and contractual safeguards in place with commercial LLM providers. Impact and Future Outlook: The conclusion reiterates GenAI’s revolutionary potential in legal workflows and its capacity to make existing processes more efficient. The authors express enthusiasm for the future, envisioning a legal practice landscape redefined by the adoption of advanced AI tools. |
Please use the PDF reader with the arrows at the bottom of the window below to advance the pages and read the entire white paper or download the PDF here.
GenAI-for-Smart-People-2
Assisted by GAI and LLM Technologies per EDRM GAI and LLM Policy.